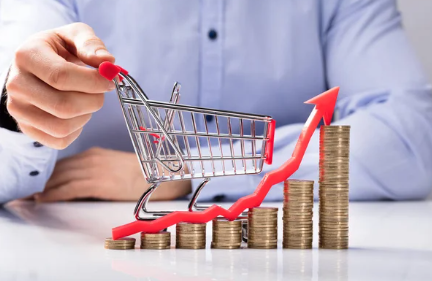
Commodities | Global | Monetary Policy & Inflation
Commodities | Global | Monetary Policy & Inflation
and enjoy exclusive professional features such as in-depth analysis, insightful op-eds, and more.
Already have Macro Hive Professional account? Log in
Spring sale - Prime Membership only £3 for 3 months! Get trade ideas and macro insights now
Your subscription has been successfully canceled.
Discount Applied - Your subscription has now updated with Coupon and from next payment Discount will be applied.