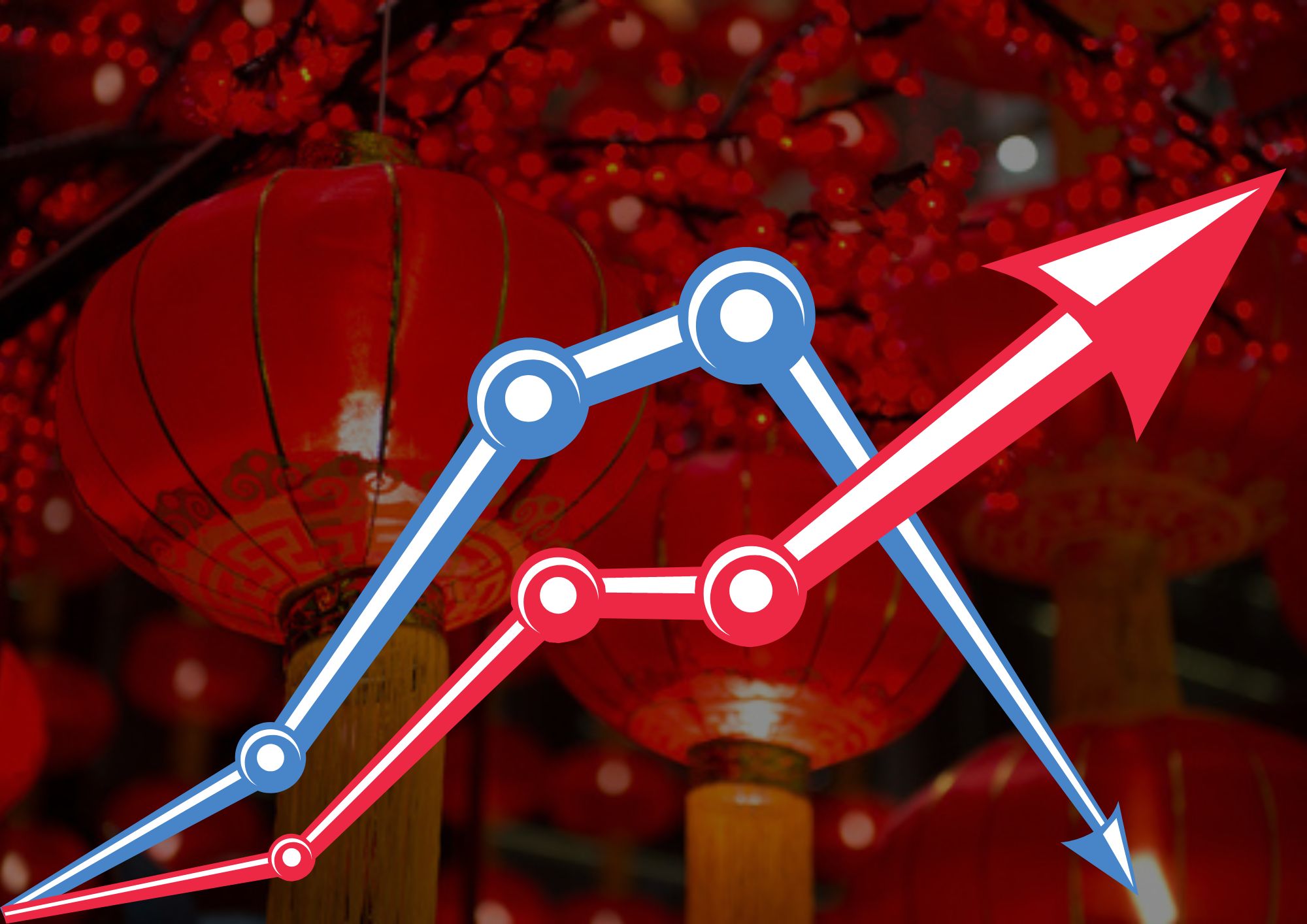
Asia | China | Emerging Markets
Asia | China | Emerging Markets
Investors, researchers and policymakers have an insatiable appetite for high-frequency macroeconomic data. It can provide more timely interventions, more profitable trading strategies, and richer economic analyses. The problem, however, is that such data is fraught with issues, such as volatility, irregular periodicities, outliers and more.
To minimise these issues while still maximising the benefits of more timely data, a new Journal of Economic Modelling paper combines both high- and low-frequency variables in the same system. And, in the context of China, the authors show that this mixed frequency model can do a better job of real-time monitoring and forecasting than more traditional lower-frequency indices, like the CEMAC published by NBS.
This article is only available to Macro Hive subscribers. Sign-up to receive world-class macro analysis with a daily curated newsletter, podcast, original content from award-winning researchers, cross market strategy, equity insights, trade ideas, crypto flow frameworks, academic paper summaries, explanation and analysis of market-moving events, community investor chat room, and more.
Investors, researchers and policymakers have an insatiable appetite for high-frequency macroeconomic data. It can provide more timely interventions, more profitable trading strategies, and richer economic analyses. The problem, however, is that such data is fraught with issues, such as volatility, irregular periodicities, outliers and more.
To minimise these issues while still maximising the benefits of more timely data, a new Journal of Economic Modelling paper combines both high- and low-frequency variables in the same system. And, in the context of China, the authors show that this mixed frequency model can do a better job of real-time monitoring and forecasting than more traditional lower-frequency indices, like the CEMAC published by NBS.
The monitoring of economic cycles has been a key research topic for over a century. Examples range from Stetson’s Harvard Index in the early 1900s to the OECD’s Prosperity Index nearly 100 years later. Their aim has always been to help us understand the length and asymmetry of cycles (up vs down periods) and movements along cycles.
Until the early 2000s, however, such indices were only put together with variables of the same frequency. That is, common factors could only be extracted from economic indicators published on either a quarterly or monthly frequency.
For example, you could learn about the US’s economic cycle by isolating a common signal from monthly non-farm employment, disposable income, industrial production and trade sales. But you could not add one of the most important macroeconomic variables – GDP – because it is published quarterly.
Thankfully, these early Dynamic-Factor (DF) models can now include variables of mixed frequencies. These are known as MF-DF models, which are popular tools for extracting economic cycles. They can also be augmented with Markov-Switching (MS) models, which monitor movements through the different stages of economic cycles.
And so, we are left with a Mixed-Frequency, Dynamic-Factor, Markov-Switching (MF-DF-MS) model that does a good job of finding economic cycles in very rich macroeconomic data; it also helps us monitor where we are in those cycles.
To construct a good macroeconomic monitoring system, you need leading, coincident and lagging variables. In China, the China Economic Monitoring and Analysis Centre (CEMAC) of the National Bureau of Statistics (NBS) has done this since 1999. It uses monthly data on various indicators, such as the YoY growth rate of GDP.
The authors set out to beat this benchmark low-frequency monitoring system from Q1 2012 to Q1 2019 by including higher frequency data. Specifically, they use daily internet keyword search data from the Baidu Search Volume Index (BSVI) – the largest web search engine in China.
Their inclusion of this data has the following steps. First, they collect daily search volumes on 177 keywords. These keywords describe macroeconomic conditions and trends, perceptions and key drivers of the macroeconomy or reflect macroeconomic repercussions.
Next, they take the monthly average of these daily search volumes to obtain the monthly search volumes, which they can then match with the benchmark CEMAC index. They then normalise the search volumes to eliminate the effect of internet development since 2012. Finally, they seasonally decompose using LOESS and take YoY growth rates.
To identify the most important keywords, they check the correlation in the growth rates of search volumes against the benchmark index. This leaves 52 highly correlated keywords. Next, they categorise these remaining words into leading, coincident and lagging indicators based on the months where the correlation is strongest.
Leading keywords are those on the Deposit Reserve Ratio, Real Estate Market and Financial Reform. The first two are negatively related to the benchmark, meaning greater search intensity regarding the real estate market usually indicates a worsening economic climate. This is perhaps unsurprising given exceptionally large size of China’s real estate market, which accounts for over a quarter of GDP – and which is under considerable strain. The full list of keywords is below (Table 1).
Next, the authors merge the more traditional low-frequency data (Table 2) with their daily keyword search volume data. This obviously creates a lot of missing values, so they treat the quarterly and monthly observations as the last day of the quarter or month, then set the other daily values equal to zero.
For the econometricians out there, missing values can be skipped in state space models, which is how the paper’s MF-DF-MS model is expressed. It means common factors between your variables can still be extracted even if there are a lot of zero values.
There are two steps to creating the paper’s China economic prosperity index.
In the first, the authors create three indexes (leading, coincident and lagging) from the high-frequency search data. They call these ‘daily public opinion indexes’, and these turn out to be highly correlated with the monthly CEMAC index (Chart 1). It implies that changes in public opinion tell a similar story about China’s economic cycle to official low-frequency economic data (but at a daily frequency!).
The next step is combining the daily opinion indexes with the low-frequency data to get the ‘daily prosperity indexes’. Here again, the results closely match the official monthly CEMAC index (Chart 2). Importantly, though, the daily prosperity indexes add richness to the economic cycle monitoring because they are calculated at a higher frequency.
According to the authors, the creation of a daily monitoring system can tell us the following about China’s economic cycle:
The authors consider these characteristics alongside all three prosperity indexes showing downward trends in 2019. This led them to conclude in 2020 that China was about to undertake a long period of medium-to-low growth. With the pandemic distorting that prediction, perhaps the lower growth in 2022 will persist for some time.
The paper is a case study for using high-frequency data alongside lower-frequency macroeconomic variables to identify nuanced traits that may otherwise be overlooked. And, rather than choosing alternative data ad hoc, the authors maintain a close connection to traditional data by demanding high correlations between the two.
In China’s case, where higher-frequency data is always hard to come by, such an analysis appears useful. That said, the paper’s methodology still relies on notoriously smooth official data. There are perhaps easier ways of monitoring China’s economy. We publish our own China growth tracker that predominantly uses commodity prices.
Spring sale - Prime Membership only £3 for 3 months! Get trade ideas and macro insights now
Your subscription has been successfully canceled.
Discount Applied - Your subscription has now updated with Coupon and from next payment Discount will be applied.