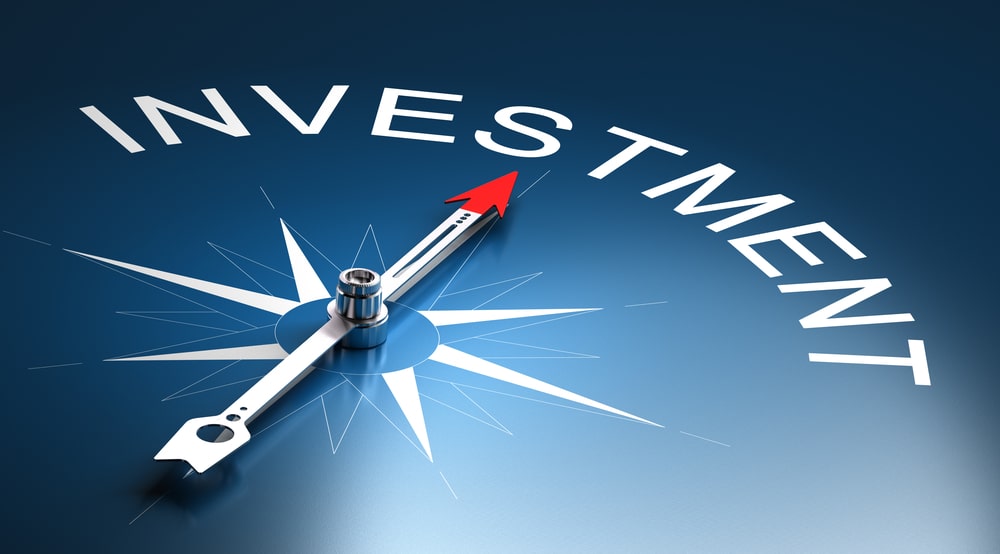
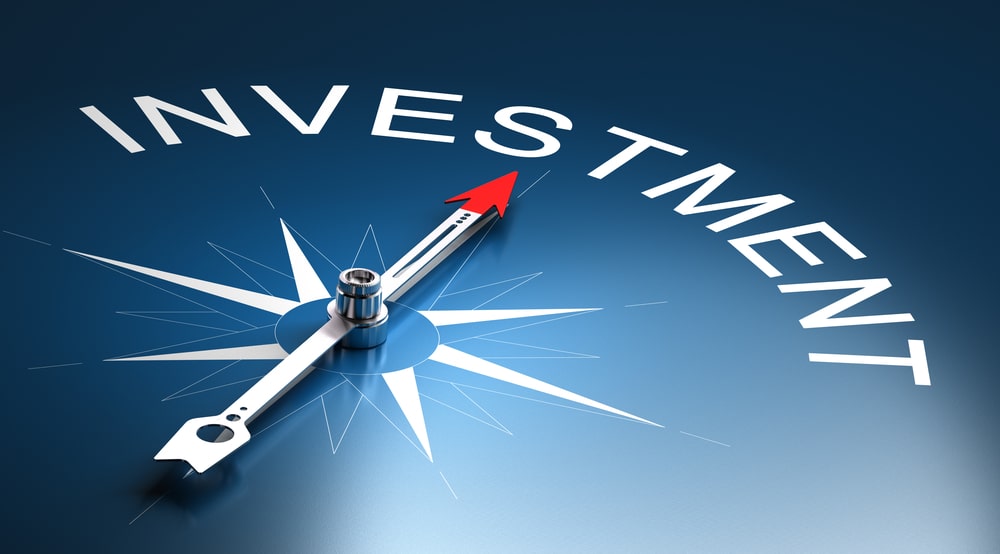
This is an edited transcript of our podcast episode with Deepak Gurnani, published 2 June 2023. Gurnani is the Founder and Managing Partner of Versor Investments – $2bn quantitative investment firm. Deepak is the former CIO of Investcorp’s Hedge Fund Group and was one of the founding members in 1996. He was also a member of the Management Committee there. Deepak retired from Investcorp in March 2013. Prior to Investcorp, Deepak Gurnani spent six years with Citicorp. In this podcast, we discuss why quantitative over discretionary investing? How to overcome data-mining and over-fitted backtests, the best way to implement trend following strategies, and much more. While we have tried to make the transcript as accurate as possible, if you do notice any errors, let me know by email.
This article is only available to Macro Hive subscribers. Sign-up to receive world-class macro analysis with a daily curated newsletter, podcast, original content from award-winning researchers, cross market strategy, equity insights, trade ideas, crypto flow frameworks, academic paper summaries, explanation and analysis of market-moving events, community investor chat room, and more.
This is an edited transcript of our podcast episode with Deepak Gurnani, published 2 June 2023. Gurnani is the Founder and Managing Partner of Versor Investments – $2bn quantitative investment firm. Deepak is the former CIO of Investcorp’s Hedge Fund Group and was one of the founding members in 1996. He was also a member of the Management Committee there. Deepak retired from Investcorp in March 2013. Prior to Investcorp, Deepak Gurnani spent six years with Citicorp. In this podcast, we discuss why quantitative over discretionary investing? How to overcome data-mining and over-fitted backtests, the best way to implement trend following strategies, and much more. While we have tried to make the transcript as accurate as possible, if you do notice any errors, let me know by email.
Introduction
Welcome to Macro Hive Conversations with Bilal Hafeez. Macro Hive helps educate investors and provide investment insights for all markets, from equities, to bonds, to FX. For our latest views, visit macrohive.com.
Before I start my conversation, I have three requests. First, please make sure to subscribe to this podcast show on Apple, Spotify, or wherever you listen to podcasts, leave some nice feedback and let your friends know about the show. My second request is that you sign up to our free weekly newsletter that contains market insights and unlocked content. You can sign up for that at macrohive.com/free. All of these make a big difference to me and make the effort of putting these podcasts together well worth the time. Finally, and my third request, is that if you are a professional, family office or institutional investor, do get in touch with me. We have a very high-octane research and analytics offering that includes access to our world-class research team, our model portfolio, trade ideas, machine learning models, and much, much more. You can email me at [email protected] or you can message me on Bloomberg for more details.
I’d also like to thank the sponsor of this episode, Kalshi. Kalshi is the first fully regulated financial exchange that allows you to trade a new type of asset class, event contracts. With event contracts, you can trade directly on a variety of economic and financial factors, including what inflation will be, how federal interest rates will change, whether the debt ceiling will be raised, or if TikTok will be banned.
Kalshi has hundreds of contracts including more traditional assets like contracts on the S&P and Nasdaq, as well as FX markets. Kalshi’s event contracts can help you protect your portfolio by hedging against specific risks or allow you to profit from being right about where the world is heading in targeted ways. You can add this new innovative form of trading by signing up for Kalshi at kalshi.com/macrohive, and you can claim a $15 credit now. And again, that’s kalshi.com/macrohive to get a $15 credit to set up an account with Kalshi.
Now onto this episode’s guest, Deepak Gurnani. Deepak is the founder and managing partner of Versor Investments, a $2 billion quant investment firm. Deepak is the former CIO of Investcorp, Hedge Fund Group and was one of the founding members in 1996. He was also a member of the management committee there. Deepak retired from Investcorp in March 2013. And before Investcorp, Deepak spent six years with Citi. Now onto our conversation. Greetings, Deepak, it’s great to have you on the podcast show.
Deepak Gurnani (02:30):
Thank you very much for inviting me. I look forward to it.
Bilal Hafeez (02:33):
Now as always, before we go into the meat of our conversation, I do like to ask my guests something about their backgrounds. Can you tell me something about what you studied at university, was it inevitable you’d end up in finance, and some of the career highlights that you’ve had up until this point?
Deepak Gurnani (02:49):
Sure. My education was primarily in India. I was fortunate, I got a really good education. I did my engineering initially at the Indian Institute of Technology in Delhi and then followed that with my master’s in business from the Indian Institute of Management Ahmedabad.
I think what is sort of common throughout, now that I look back, it was always a very strong interest in computer science, mathematics, and finance. In that regard, probably it was inevitable that I would enter finance and quantitative investing. That’s really what I’ve done for a long period of time.
But I recall that even while I was at college, I had written software to trade equities using trend following, developed models for convertible boards. Nothing quite like what we do today, but certainly early days itself. And then I worked initially as a software professional for five years or so at Citigroup. I started in India, but I was based primarily in Europe at multiple locations ending up in London.
Bilal Hafeez (03:55):
And that was on the software side rather than the trading or portfolio management side or anything?
Deepak Gurnani (03:59):
Yes. That’s actually where I got my first formal exposures to investment and risk management, as a software professional, but focused on investment, risk, and trading activities. Given my background, that was something of tremendous interest and I learned a lot in those five years.
Then I joined Investcorp, and very quickly, I was the head and CIO of the Hedge Funds Group based here in New York. I was also a member of the management committee. And it was really in Investcorp that I got exposed to all aspects of hedge fund investing, right? So the different strategies, asset allocation, risk management, I published extensively during that period. But I think again, what is the sort of common throughout the stay? It was a very strong focus on quantitative investment strategies, and that too on absolute return strategies. That has been a concern.
Then I set up Versor in early 2014 to focus on quantitative investment strategies. What we do today is only quantitative investment, we focus on absolute return strategies across multiple asset classes. We are very scientific hypothesis-driven framework that we use. And we offer both hedge funds, which is where the bulk of our assets are, as well as risk premium products. So that’s sort of been the evolution from education all the way to where we are currently.
Bilal Hafeez (05:28):
And where’s the name Versor come from?
Deepak Gurnani (05:31):
So it’s actually very interesting. The name Versor, this is a mathematical system that is used. So this came from an internal exercise that we did when we were naming the firm, and this was one of the names that was suggested actually by my son, Nishant. He suggested the name, and the rest of the partners, we all quite sort of like that and that’s why we started.
Why Quantitative Over Discretionary Investing?
Bilal Hafeez (05:56):
Okay, no, that’s great to hear. And it’s good to have a name that doesn’t involve the word black or blue or those sorts of things in the name too.
Now, when you were earlier at Investcorp, you oversaw different strategies, discretionary, quantitative, all sorts of different hedge funds strategies as well. But now with Versor, you’ve gone down the quantitative route. One more philosophical question is why pick quantitative over discretionary? Or why pick just quantitative? You could have gone for a hybrid approach, 50% quantitative, 50% discretionary, or you could have gone for a continental approach where you kind of mixed the two together. So why quantitative?
Deepak Gurnani (06:39):
For me, personally, it was a relatively easy decision. I think it goes back to my strong interests pretty much from my education and throughout my career in computer science, mathematics, and finance. If you look at the intersection of those interest areas, it lends itself to quantitative investing.
I’ve always had a bias towards quantitative investing. I think you very correctly said that, at Investcorp, I did have exposure to both quantitative and discretionary strategies. And I want to say that I have tremendous respect for discretionary traders and managers, but I think just given my strengths, it made sense.
I actually found that combining the two, at least from my perspective, was suboptimal. When we set up Versor, I think the first early decision was we would do quantitative strategies only. We would not do a hybrid approach, we would not use some of the other sort of approaches that you mentioned, right? We’d focus on quantitative.
And look, jokingly, seriously, I always say I have never seen a computer get emotional or lose focus or lose consistency. Quantitative investing, it is I guess something that I and the other partners here are very comfortable with and that’s why we sort of chose that approach. Having said that, again, as I said, I have tremendous respect and both these approaches I think will coexist and the debate will also be always there.
How to Overcome Data-Mining and Over-Fitted Backtests
Bilal Hafeez (08:06):
No, you’re right, it always will be. And in the end, you have to play to your strengths and clearly you have a really quite impressive pedigree on the quantitative side. One perennial challenge with quantitative approach is the problem of data mining where you basically backtest the data, you torture the data and the results always look fantastic. How do you get over that kind of fundamental or original sin or quant approaches?
Deepak Gurnani (08:29):
That’s a fantastic question and I think that is sort of at the cross. When you are in quantitative investing, the discipline that we use is to be hypothesis-driven. We typically start with the hypothesis and let the data either support or go against or reject the hypothesis as compared to essentially saying, ‘Okay, here’s a bunch of data, find some patterns, et cetera, that might be helpful in identifying the strategies.’ Again, there are folks who probably do that successfully, not for us.
Our approach is we are way data-driven, we are also hypothesis-driven. And we start with the hypothesis, we use a scientific approach, we use several modern statistical techniques and come up with our strategies. I think one of the pitfalls again within the statistical techniques is overfitting, right?
So essentially, data mining and overfitting whereby you are essentially using the same data set to estimate the model and then test whether the results look good or not. And when you do that in sample, invariably, the results will look good and only for the results to not look as attractive on an out-of-sample basis, or worse, lose money on an out-of-sample basis.
We spend a lot of time on the statistical techniques that we have developed, adapted and used, essentially separate out the data that we use for estimating and the data that we use for evaluating. Again, I’m generalising and oversimplifying, but we are very conscious of that and it always gets interesting that when you get young new professionals, to see them sort of go through the process of first looking at the results, getting over excited, thinking that they’re doing really well and then sort of figuring out some of the common pitfalls with the data mining and overfitting. And I guess getting that right is a very important prerequisite to be successful in this region.
Bilal Hafeez (10:37):
I’ve done a lot of work in the quantitative space as well over the years and one thing I found frustrating is if you do it properly and you show your backtest, the returns won’t look as good as somebody who’s overfitted the data. Yet when investors look at the chart with a line going up dramatically, something happens in their mind where they get drawn to a P/L chart that looks really, really impressive even though it’s been data fitted and overfitted.
I mean, how do you convince investors that maybe your returns may not look as good as some other simulated returns elsewhere, but your approach is more robust? I mean, how do you get them over the line to look at the data in a more realistic way?
Deepak Gurnani (11:15):
So I think we’ve been somewhat fortunate that the industry has evolved as well. What I mean by that is our client base is the large pensions and institutions globally, the staff that we work with, the professionals are very experienced in quantitative techniques and understand that you cannot rely on backtests and simulations really for making investment decisions.
It is inevitable. Some amount of use of backtest and simulations are inevitable as sort of the part of the process, but I think we tend to share our healthy scepticism that we have. I would say that the backtest and the simulations that we generate are predominantly for internal use for us to evaluate the strategies and determine which signals or forecast or strategies will go into production and live versus not.
And in that regard, there’s no incentive for us to look at impact. I think my partner here, Ludger Hentschel, who has researched with me, says he’s never seen a bad backtest. You never sort of share a backtest that doesn’t look good. I guess that tells you a little bit sort of what our mindset with using backtest and simulations.
The Best Way to Implement Trend Following Strategies
Bilal Hafeez (12:34):
Yeah. Okay, so let’s talk about one of the most popular types of quantitative strategies, which is trend following or momentum-type strategy. Obviously, the essence is if the price is going up or down, you follow that trend.
Now I guess the first question I have for you is, as you said, taking a hypothesis-based approach. Why do you think trend generally tends to work in multiple different markets? I mean, what’s the theory behind why trend would work? Because obviously, we’re taught at school in theory, efficient markets hypothesis that past prices shouldn’t tell you anything about future prices and so on. How do you square that circle?
Deepak Gurnani (13:11):
So I think if you go back to the basics of trend following and see how does trend following make money or how does it sort of generate the positive convexity, trend following signals essentially identify the trend, whether the trend is up or down. If you correctly identify the trend, if the trend is up, you take a long position, make money as the markets rise. If the trend is down, you take a short position, and again, make money when the markets are falling.
And the extent to which you can capture both the upside move as well as the downside move in the markets, which is a measure of, statistically, we refer to that as the convexity, I think it gives you a measure of that particular strategy or that particular trend following signal. I think we can certainly also demonstrate, we wrote a paper on that, it’s on our website on how trend following has evolved.
And historically, what we have seen is trend following has an attractive property of strong returns as well as positive complexity. And that’s hard to get, and as a result, trend following has been popular and fits well into institutional portfolios. We have a successful trend following strategy as well, but at the same time, I think the point that we made in the paper is that not all trend following strategies are the same and we have seen some deterioration in properties of trend following, I would say, over the last 10-plus years or so.
Bilal Hafeez (14:37):
And so when you say convexity, just to be clear for our listeners, what you mean is that the gains you make when the strategy is making money is much larger than the losses? So you get this kicker there, is that what you mean in terms of convexity?
Deepak Gurnani (14:49):
Ideally, to demonstrate positive convexity, you want to have positive data in up markets, right? It could be equity markets, it could be bond markets or commodities. You want to have positive beta in up markets to make money and negative beta in falling markets to make money in falling markets. And investors obviously value both positive beta and negative beta, but specifically negative beta and down markets is very attractive from an institutional portfolio point of view. The betas between the positive and the negative beta, the extent of that shift between positive to negative data is a measure of the convexity.
Bilal Hafeez (15:36):
Okay, yeah, understood. Now you did say that over the past, say, 10 years, there’s been a deterioration in the performance of momentum strategies, on what should be clear amongst funds that follow these strategies both in terms of performance and convexity and so on. Why has that been the case?
Deepak Gurnani (15:53):
So the background to this, we published a paper back late in 2020, I think we formally published it in 2021. And what was interesting, what we found was that in the first quarter of 2020, trend following index, so I’m referring to the index here, did not do as well. And we saw a big divergence between how our internal trend following strategy performed, which did really well during that period and sort of then got our interest going as to what is going on. At that point, we had also seen that there had been more than a decade of low returns to trend following.
When we embarked into the strategy, we found something very interesting. And what we found was that when you look at the kinds of signals that are embedded in the index returns, now the index is a combination of multiple managers, clearly there are about 10, 12 managers in that specific index that we studied. And what we found was that the proportion of investments in the longer-term signals had increased over time, and the longer-term signals have consistently generated poorer convexity. The short-term signals have the most positive convexity, but the transaction costs are much higher.
So an ideal strategy in our view is to combine short-term, medium-term and long-term trend following signals and that sort of works well over time. But we found that the larger funds, presumably because of growth in assets or other way, we found it very difficult to employ short-term, medium-term trading strategies because of the cost involved and gravitated towards the longer-term signals and that resulted in significantly lower convexity. And now, in the recent period, 2022 and parts of 2021, trend following has worked very well and that’s because the long-term signals have worked extremely well over that period. So our key conclusions from the paper are sort of unchanged.
I think the second important point that we make in our paper is that trend following is one strategy to achieve positive convexity, to the extent that the investor objective is to have positive convexity in their overall portfolio returns. We do believe that there are non-trend signals and strategies that can be profitable. For example, we demonstrate in the paper, one of the strategies that we run is a cross-sectional strategy in global equities, equity indices to be precise, we refer to it as the Global Equity Tactical Trading strategy. Historically, it has a significantly higher convexity than trend following as an example. So those were the two real conclusions. One is-
Bilal Hafeez (18:39):
And when you say cross-sectional, what do you mean there? Do you mean you compare the performance of different equity markets, the returns, and rank them and then trade that momentum?
Deepak Gurnani (18:49):
Yeah. So when we say trend following, trend following makes money by essentially betting on the direction of the market, right? Trend following invest in equities who are either net long equities, net short equities, net long fixed income, net long or net short commodities.
Cross-sectional strategies essentially are strategies which do not take a bet on the direction of the market. The strategy that I was referring to, the Global Equity Tactical Trading strategy essentially ranks exactly the way you mentioned, but it does not rank based on momentum alone. It uses 30 different forecast models from micro-models which are looking at microstructure of the markets to using economic data.
I think the key there is that once you have multiple models and your forecast models are designed to make money, as markets become volatile, that lends itself to positive convexity. So if you have forecasts that do well when markets are volatile, even cross-sectional strategies, designed correctly, can generate significant positive convexity.
And that’s what we elaborate on further within our paper that there are two approaches. One is within trend following, it’s very important to mix short-term, medium-term and long-term, and avoid the temptation to just go towards, for example, long-term signals. And secondly, you can further improve the overall investor portfolio by having other complementary strategies that also exhibit positive convexity.
What Is Merger Arb and How it Works
Bilal Hafeez (20:27):
Yeah, that makes a lot of sense. What you’ve essentially said there is that many trend following kind of funds have drifted towards these long-term strategies and what they’ve sacrificed then is this positive convexity where you have attractive beta in down markets as well as up markets. And then there’s ways around this by, as you’ve done, have a range of horizons, the trend and then do this cross-sectional type strategies as well. And you’ve published a paper on this, very kindly as well, so people can refer to the paper where you go into much more detail to show the performance.
Okay, so that sounds great. Yeah, let’s move away from trend to a completely different type of strategy, and I understand you do the strategy as well, mergers and acquisitions, M and A arbitrage strategies. Maybe first of all, could you just describe what we generally understand by M and A arb strategies. And then secondly, and I have to reflect my own ignorance, I didn’t realise there was a quantitative approach to M and A arb. For some reason, I always thought that it’s being a discretionary strategy. So if you could answer those questions, it’d be great.
Deepak Gurnani (21:29):
Sure. Merger arbitrage, in the most sort of basic sense, when a merger is announced, let’s say if the target company quotes at, let’s say $70, I’m just using hypothetical example here, and the acquirer comes in and announces that, ‘We like to take over the company for a $100,’ you’ll immediately see the stock price increase from $70, not quite $200, but at a discount $200. It could be at $95, $96, $98 or it could be $90, depending on the nuances of that particular transaction.
And merger arbitrage strategy essentially looks to earn that premium once the deal is announced. So it’s important that classic merger arbitrage gets involved when the strategy is announced and not before that. And once the strategy is announced, there are a lot of reasons, some academics otherwise that we have seen why the premium exists, but the idea is to capitalise on that.
The risk though is that if the merger does not conclude for any reason, whether that’s due to regulatory reasons, it’s due to other considerations, the price could fall back to $70 or even lower. Imagine if you bought it at in the $90s and the deal breaks and the price goes back to the $70s, you end up losing a lot of money. The merger arbitrage manager essentially looks to invest in a portfolio of deals where typically you’ll have $30, $40, $50 deals at any point in time, sometimes more, sometimes a bit less depending on the deal flow, and look to run a diversified portfolio and weight the deals based on an assessment of whether the deals will succeed or whether that would fail. That’s at a basic level, that’s the kind of premium that you’re looking to earn from merger arbitrage.
Bilal Hafeez (23:27):
And typically, is this a discretionary strategy or is it quantitative or both?
Deepak Gurnani (23:32):
Historically, I would say this strategy has mostly been a fundamental strategy. When I first started working in merger arbitrage and researching into merger arbitrage in the mid-to-late ’90s, it was essentially a fundamental strategy.
And what was really interesting was that when you looked at portfolios of multiple merger arbitrage managers, they were near identical portfolios. They are not quite exactly the same, but you could see the same kind of deals within the portfolio, more or less sort of size based on liquidity and market capitalization. There was some differences. And every time the deal broke or terminated, it would impact all the portfolios and you would see losses.
That sort of got my interest going and said that maybe there’s a quantitative approach to be able to capture that. I actually first worked on it since 2000, published a paper back in 2008, 2009. Again, what we do today is very different from the initial work that we did. And the strategy evolved and we very firmly believe that quantitative approaches to merger arbitrage work quite well. We have been successfully running the strategy since 2015.
Bilal Hafeez (24:49):
Okay, great. And in terms of which deals you include, number one, and also how do you go about assigning a probability to success in the deal quantitatively?
Deepak Gurnani (25:00):
So essentially, what we do is we first started by building a historical database of 4,000-plus deals. We have every single deal announced globally since 2000. It goes through a significant amount of human vetting where we get the data from multiple sources, somebody reads the press release. We have a very good proprietary database of 4,000-plus deals with all the different deal characteristics as well as the final outcome. We then use advanced machine learning methods using the data to be able to come up with forecast models for a variety of forecasts.
There is a difference in the way we sort of approach merger arbitrage. Most people, when they look at merger arbitrage, they look at it as a binary model whereby either the deal succeeds or it terminates. If it succeeds, you make some money. If it terminates, you end up losing the significant downside.
We have a modified approach. What we actually found is that, let’s say on average, 7% to 8%, if you include globally, 9% of the deals fail on average. It changes over, it varies over time. The remaining 91% of the deals, what is interesting is a significant portion of those, about 15% out of those 91% left of the deals that succeed actually get a competing offer or an improved bid and you end up making more money than the initial spread on the bid.
And in addition to focusing on the downside, which is very critical, we also focus on determining if there will be a competing bid or an upward revision to the price that is being offered. I can confirm by talking to different desks and other investors that very few, if any of the merger arbitrary managers, really focus on that. Almost everybody focuses on the downside.
We have a variety of forecast models. We forecast whether the deal will succeed or fail. We will forecast whether there will be a competing bid, and then we forecast a lot of trading related parameters as well. Where will the spread trade on the day of announcement? What kind of trading volumes would we see? So we have what could be the tenor of the deal, whether the deal will flows in six months or nine months. The management provides some input, but again we have a multi-forecast model which will then look historically at deals having similar characteristics in an intelligent manner using machine learning techniques and make an assessment. And the sum total of those forecasts are then used to make an assessment of what do we think is the expected return, what is the risk, and we would size the deal within the portfolio accordingly.
So again, at a very simplistic level, if the deal is risky, we would tend to have smaller rates in the portfolio. If the deal is safe, we would have larger rates in the portfolio. If the deal has a higher probability of a competing bid, we would increase the weight in the portfolio. If the deal has very large downside, let’s say if a biotech firm is being acquired by a large pharma, there’s a good chance the deal will go through, but if the deal does not go through, the biotech company could have very significant downside. We need to take that into account as we size the deals within the portfolio.
We’ve been using it since 2015 and we have had very good results. And we firmly believe that it’s a very good approach to merger arbitrage investing.
ESG Component of Merger Arb
Bilal Hafeez (28:39):
And I’ve read one of your recent papers which showed actually another interesting characteristic of these strategies, at least in the way that you implement them, it’s ESG scoring or component to it. There’s an ESG angle to this, which I didn’t ex-ante. I wouldn’t have thought there would be anything to do with ESG here, but could you talk more about what your findings were in terms of your merger arb strategy?
Deepak Gurnani (29:02):
That was a very interesting project. And as we worked on incorporating and assessing impact of ESG on all of our strategies, on merger arbitrage, there is almost no research out there in terms of how ESG impacts merger arbitrage. There’s a lot of research on how ESG impacts stocks in general and stock portfolio but not so much on merger arbitrage. Again, we embarked on that analysis internally.
One of the advantages we had was we have more of our forecast, our database of 4,000-plus deals that we have, so essentially retrieved ESG scores. I would say although we have data since 2000, we have very good quality data over the last 5 to 10 years. Remember, prior to that ESG was not as much a focus so there’s not as much sort of coverage of the companies.
And what we found to our real interest was that on average, we saw a 50% increase in ESG scores for the Versor Merger Arbitrage strategy. So we felt that it certainly warrants looking into more detail because when we look at the sheer magnitude of improvement, it surpasses any other investment strategy, at least that we are aware of, where in most cases, the investment approach is to invest in companies that have good ESG scores, hold them over a period of time and literally hope for the best, that leads to an improvement.
In merger arbitrage, given that the merger event is one of the most significant events in the life cycle of a company because it gets acquired or it acquires another company, we actually can demonstrate that associated with the merger event. If you measure the scores a year before the merger event and one year after the merger event is completed, it can be attributed to the merger event itself. There’s an improvement of 50%.
Bilal Hafeez (30:58):
Why would it lead to a big increase in the ESG score after merger event?
Deepak Gurnani (31:03):
So I think in general, if we compare that to a naive merger strategy, the improvement is much less but nevertheless it is there. When I speak about numbers like 50% improvement for the Versor Merger Arbitrage strategy, there is about 25%, 30% improvement for the naive merger arbitrage strategy as well.
When we go into the details, what we see is that typically the target companies that get acquired have a lower score than the sector average. Again, the numbers that I’m quoting are, with respect to the sector, averages. And what we see is that because of the increased focus on ESG, there is an upward trend in ESG scores for all the sectors, when you combine all the companies within the sectors.
However, what we do find is that the companies that get acquired, the target companies, to start with have slightly lower scores as compared to the sector average, the acquirer company, which typically is a larger company, let’s say has scores closer to the sector average, but the combined company has significantly higher scores as compared to the average for that sector. The merger activity acts as a catalyst and we see that certainly in the last five years or so that whether explicit or not, ESG considerations are becoming a large part of the merger rationale and thesis. Again, as I said, whether explicitly stated or not.
So what we do as a part of the merger strategy is that we carefully measure the ESG scores of the companies involved and actually make an assessment of where do we think the combined companies will be one year after completion of the merger. Again, we are able to study that based on the historical database that we have. And as a result, all of the things being equal, we would overweight those companies where we think there would be a significantly higher improvement in ESG scores. As a result, we are able to achieve higher scores than just the sector average, which also is increasing at a reasonable rate.
Why Value Investing Has Underperformed
Bilal Hafeez (33:23):
And that is really quite a remarkable finding actually and something that’s quite novel, I think, that you’ve discovered. Hopefully, people hear more about this.
Now I did want to, we are talking about quantitative strategies, so I have to ask you about equity value. Equity value for years and years and years, people have been saying, ‘It should come back, it should come back,’ but it’s that horrible 10, 15 years. What’s your thoughts on the value factor? It’s been a tough one for systematic or people to trade factors.
Deepak Gurnani (33:53):
So I want to first point out that the way we implement value, again, we’re not a value manager per se industry. We do use value strategies as a part of one of our quantitative equity strategy. And there are essentially two differences between how we implement value versus the academic approaches, and I think that goes to at least a part of the comment that you had in your question.
I think the first is unlike academic approaches which tend to use a single value factor, we have multiple value factors. We look at earnings, we look at balance sheet, I invoke the price, we look at EBITDA, we’ll adjust for growth, we’ll adjust for a variety of things, so we have multiple value factors. That’s one difference.
The second difference is that when we invest in value strategies, we tend to do on a long, short basis where we are sector neutral and that’s a very crucial difference. Most folks when they refer to value and growth within the equity markets refer to sectors. Technology is a growth sector, right? Financials is a value sector and so on. Our investment, I think it’s relatively common within the quantitative equity managers to run it on a sector neutral basis, not everybody does that, I think quite a few do that, we certainly do that. So as a result, our portfolios are long and short technology, long and short financials, long and short energy. When we say we are focused on value, we are not short technology, we are long technology and short technology and we are residual, no residual exposure. I think that’s a crucial difference.
So when there is a mention that value strategies have had a very difficult 10 to 15 years, that’s more when you refer to the more of the naive academic value definitions. When you just look at, for example, the Russell Growth versus value indices, that observation has been correct, but the more sophisticated versions of value have actually performed well except over two different periods and I’ll elaborate.
So the background to this paper was really the underperformance of value styles over the 2018 to 2020 period. Clearly, in 2020, value suffered significant losses. And what was interesting is that the 2018 to 2020 period, you saw underperformance for value across the different styles. It did not matter whether you had one value factor or you had multiple value factors. It did not matter whether you were sector neutral or not. In fact, I think it did matter. Sector neutral, that’s less money than folks who were essentially just naked short technology being a growth sector and we saw that value lost money globally. So that got our interest going.
The last time we had seen that happen was around the 1998 to 2000 period. Towards the end of the technology boom, we had seen that value had underperformed significantly over that period, but then as things normalised within the markets, we saw the tech boom burst, saw several years of outperformance from the value factors.
And that’s why when we wrote this paper in 2021, we made the link back to the tech bubble period and said that we are seeing that the differences between the cheap stocks that became cheaper and the expensive stocks that became even more expensive, the difference got magnified to the same levels and, in some cases, actually even surpassed what we had seen at the end of the technology boom. We made the claim in the paper that while it may be difficult to get the timing correct, we did believe that as things normalised, that we would see a return to value strategy. Again, we are not a value manager per se, although we do use value.
Our strategies that do focus on value did benefit as we saw some return to value strategies, I would say over the last couple of years or so, certainly in 2021 and 2022. And what could be the trigger for that, whether it was higher interest rates that led to investors focused more on profitability of the company than which companies were going to make profits and so on. I think it did favour value stocks and we saw that for a period of two years. Again, it was not in a straight line, it was volatile. We do see that wild spreads have come in somewhat, but the opportunity continues to be there.
How to Combine Strategies
Bilal Hafeez (38:40):
Yeah, that’s great. So you have so many, many strategies that you deploy, trend, momentum, or factor models, risk premium models, the merger arb models, and so many others. What’s your approach to combine them all together? Do you have a favoured sort of portfolio optimization approach?
Deepak Gurnani (38:56):
So we do use portfolio optimization at multiple levels. Some approach which is sort of an optimization-based approach is used within each of the strategy for portfolio construction. In every strategy, whether it’s merger arbitrage or our Global Equity Tactical Trading strategy or a foreign equity strategy, there’s always a trade-off between risk, return, liquidity, transaction costs, and controlling for overall exposures. We do use optimization methods.
Historically, we have not focused as much on a single multi-strategy that combines these products. We haven’t done that. Again, the background to that is our investors, which are the large institutions globally, tend to do their own constructions and asset allocation to the different strategies and are interested in picking the best equity tactical trading strategy or a merger arbitrage strategy. Having said that, we are looking at potentially combining the different strategies that we run into a multi-strategy, although we haven’t formally launched that as yet.
My partners and I have significant experience doing that from our prior work that we did, for example, during my tenure at Investcorp and my other partners at the various places that they worked with over time. We were pretty confident in the way it needs to be done, that particular optimization. I think the thing that one needs to be most careful when you’re combining the strategies is not to just focus on a mean variance risk-return, just kind of an optimization, but to focus specifically on convexity and performance in different environments.
In that regard, our optimization approach is a combination of the classic optimization along with other scenario-based and other approaches that actually trade off convexity with returns and with portfolio performance in different environments. And it sort of makes it a level more complex than just sort of traditional optimization, but that’s something that we are working on and we haven’t launched it as yet. Some point this year, that is the product that we will be launching.
Thoughts on AI
Bilal Hafeez (41:18):
And currently, there’s so much talk about AI. Obviously within AI, you have machine learning which you referred to earlier, but also ChatGPT, LLM models, and so on. What are your thoughts on this AI boom that we’re seeing at the moment?
Deepak Gurnani (41:32):
So we have been using machine learning pretty much since inception at first. I think what is exciting has been the availability of large amounts of data that clearly, in the so-called alternative data, has really exploded in terms of the amount of data that is available combined with cheaper computing power. For example, we have been, even pretty much since the inception of the firm, even when we are much smaller, we have been using cloud services to get that kind of scalability. There’s a significant amount of data and cheaper computing power. The combination of that, I think, is very exciting and has enabled different investment managers to be able to use the strategies.
I would say though that it is still relatively new in quantitative investing. Even the folks I think who do use it, like we do use it, still do that somewhat cautiously and combine it with some of the other traditional approaches as well and use it for sort of localised problem solving. For example, I mentioned it within mergers, pretty much since inception, we do use machine learning methods to come up with a forecast of how the deals would operate. We do that for several of our higher frequency equity strategies. Nevertheless, there are some challenges. The challenges with ability of using machine learning to control for turnover within the portfolio, to be able to control for risks within the portfolio.
It’s an exciting field that is evolving. I think clearly some of the success that we have had is proprietary, so I prefer not to sort of go into too much more detail on that, but I think it’s exciting, but I think it’s important to recognise it’s still relatively new. There are challenges, but it is certainly here to stay. We have been making significant investments and we’ll continue to do that.
Bilal Hafeez (43:22):
Yeah, that’s great. Now I did want to ask a few personal questions. What’s the best investment advice you’ve ever received from anyone?
Deepak Gurnani (43:30):
Think about that, right? Aside from the Versor investment strategies, I think it’s going to be difficult for me to pick, within the investment strategies, we get good advice. If I keep that aside, I think there certainly are two pieces of advice that do stand out when I look back over the last several decades.
I think first is fairly simple, but I think very powerful, is I got that advice early on and I do share that with whoever cares to listen, is that always have a portion of your savings invested in passive US equities. And literally, you can do that at next to zero cost. I’d say few basis points as literally zero cost versus investment management to compliment it. Again, as I have said, all of my liquid network worth is in the Versor investment strategy, but the advice I do value and I pass on to my children and my friends who are not necessarily in finance is to always have a portion.
I think the second piece of advice that I received was regarding shorting US subprime debt in late 2006 while I was at Investcorp. I think when I look back at my career in Investcorp over the 20-year period, I think that was the most profitable investment strategy that we did as a part of the Hedge Fund Groups. I was certainly very proud of that and that’s certainly adjusted. Very different again, right? We’ve been obviously very successful at Versor investment strategy, it’s difficult for me to pick.
Bilal Hafeez (45:02):
Yeah, no, I understand. We do have students listening to this podcast as well. Question I’d like to ask my guests also is what advice would you give to students leaving university today as they enter the real world?
Deepak Gurnani (45:14):
Very recently, I heard a speaker articulate literally in a few minutes the importance of reinventing yourselves every few years. Especially when you are coming out of university, you’ve gone through a lot of education and it’s important to recognise that that’s just a start. It’s not the end, so you have to reinvent yourself. The world is changing, has always been changing, it continues to change, and to be ahead, one has to be able to adapt. In my mind, that is one.
Second, I think something that is more topical also is that to be successful in any field today, not just in investing but in any field, I think today one has to have the ability to manage and process vast amount of information. You could be in any field, just a significant amount of information and you need to manage and process that. So having some basic skills, at least in computing statistics and data management, I think will give an edge in any theory. And I would say those are the two things I would certainly say at this point.
Bilal Hafeez (46:23):
Yeah, that’s great advice there actually. And not many people have said that actually, but it makes so much sense what you’ve just said there.
What’s the best way for people to follow you if they wanted to reach out to you, if they’re interested in your strategies, and then also the work that you’ve been producing that we refer to, what’s the best way for people to connect?
Deepak Gurnani (46:40):
So we do publish literally all of our work. A lot of our work is published on our website, so to get to see what we are doing, our papers are all on the website. Certainly through website or through LinkedIn is the best way to get in touch and I look forward to that.
Bilal Hafeez (46:57):
Great, and I’ll include the links in the show notes as well so people can just click through directly to the site. So with that, thanks a lot, Deepak, it was great speaking to you. I’ve learned a huge amount and I will definitely be reading all the papers that you’ve been producing over the years.
Deepak Gurnani (47:12):
Thank you very much. It’s a pleasure. I really enjoyed the discussion. Thank you.
Bilal Hafeez (47:17):
Once again, I’d like to thank the sponsor of this episode, Kalshi. Kalshi is the first fully regulated financial exchange that allows you to trade a new type of asset class, event contracts. With event contracts, you can trade directly on a variety of economic and financial factors, including what inflation will be, our Fed interest rate changes, whether the debt ceiling will be raised or if TikTok will be banned.
Kalshi has hundreds of contracts including more traditional assets like contracts on the S&P and Nasdaq, as well as FX markets. Kalshi’s event contracts can help you protect your portfolio by hedging against specific risks or allow you to profit from being right about where the world is heading in targeted ways. You can add this new innovative form of trading by signing up for Kalshi at kalshi.com/macrohive, and you can claim a $15 credit. Again, you can sign up Kalshi at Kalshi.com/macrohive and you can get $15 credit now.
Thanks for listening to the episode. Please subscribe to the podcast show on Apple, Spotify, or wherever you listen to podcasts. Leave a five-star rating, a nice comment, and let other people know about the show. We’ll be very, very grateful. Finally, sign up for our free newsletter, at macrohive.com/free. We’ll be back soon, so tune in then.