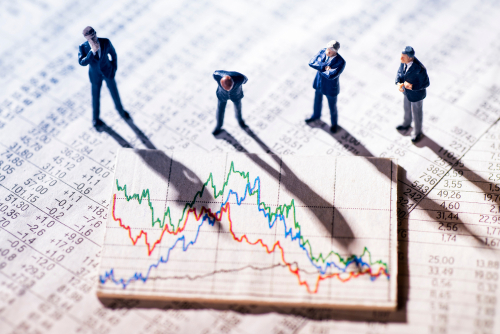
Monetary Policy & Inflation | US
Monetary Policy & Inflation | US
Momentum models, either cross-asset or single-stock, typically perform well during inflationary periods. As a result, we have seen an increase in studies examining the performances of enhanced trend-following strategies.
This article is only available to Macro Hive subscribers. Sign-up to receive world-class macro analysis with a daily curated newsletter, podcast, original content from award-winning researchers, cross market strategy, equity insights, trade ideas, crypto flow frameworks, academic paper summaries, explanation and analysis of market-moving events, community investor chat room, and more.
Momentum models, either cross-asset or single-stock, typically perform well during inflationary periods. As a result, we have seen an increase in studies examining the performances of enhanced trend-following strategies. For example, we recently showed how oil price volatility improves the performance of a single-stock momentum model.
Now, a new Journal of Banking & Finance paper compares the performances of three stock price volatility-enhanced momentum strategies. They differ in the way momentum is scaled by volatility, but all decrease crashes and lead to higher risk-adjusted returns.
Momentum strategies can be very profitable. They are also, however, prone to failures.
For instance, after bear markets, the market betas of loser stocks tend to be higher than those of winner stocks. So, when the market rebounds after a bear market, the overall negative market sensitivity of the winner-minus-loser strategy generates negative returns during the market up-movement.
Momentum returns are also sensitive to market transitions. For example, market up-movements (down-movements) that follow a bear (bull) market are associated with low momentum returns. Meanwhile, momentum returns in markets that continue in the same state are generally higher.
Volatility scaling aims to manage these crashes, according to the authors. This exploits two the empirical facts. First, returns are relatively low when volatility is high (the leverage effect). Second, factor-return volatility is positively autocorrelated in the short term.
Not only do the authors show that these strategies increase Sharpe Ratios; they are also profitable after accounting for transaction costs – something standard momentum strategies struggle to be.
The paper’s sample is 66,905 stocks from 49 equity markets. For the US, this includes all common equity stocks traded on NYSE or NASDAQ from 1926 to 2017. For countries outside the US, equity data is collected from Refinitiv for 1987 to 2017.
Both daily and monthly returns are calculated and measured in USD. Daily returns are used to construct the standard and volatility-scaled momentum strategies. And to do so, the authors require a firm to have at least 30 months’ worth of return data, otherwise they are dropped. In total, there are 8,550,324 firm-month observations.
To evaluate the risk-adjusted performances of the volatility-scaled momentum models, the authors construct factor portfolios. These include the Fama-French market factor, RMRF, size factor, SMB, and value factor, HML, and a momentum factor, MOM. For brevity, I only summarise how they create their volatility-enhance strategies.
Volatility scaling aims to manage the realised volatility of an investment strategy. The authors assess three types of scaling: constant volatility-scaling (cMOM), constant semi-volatility scaling (sMOM), and dynamic-volatility scaling (dMOM) momentum models.
cMOM adjusts the momentum portfolio to a constant target volatility level. In other words, it applies a weight to the daily returns of a momentum strategy, which in this case, is evaluated over the last 126 trading days. More info can be found here (page 11), but the weight consists of two parts:
(i) a target volatility, which can be arbitrarily set depending on the annualised volatility of monthly returns in the unscaled momentum model – say 12%; (ii) a monthly forecast of expected volatility, which is calculated using daily returns of momentum in the previous six months:
where is the squared realised daily return of momentum returns summed over the last 126 trading days. So, to get the returns of the cMOM strategy, simply multiply the daily returns of a standard momentum strategy by the weight
The sMOM strategy is the same, but only accounts for semi- or downside-volatility momentum. More info on this one can be found here (page 4), but in essence it just calculates volatility on days where daily returns in the standard momentum model are negative:
Lastly, dMOM enhances cMOM by additionally incorporating the expected strategy return. So, the dynamic weight becomes:
where is the forecasted monthly return, say 0.5%. To forecast monthly returns, they use a regression, but you could simply use a 12-month moving average filter instead.
So, to sum, the monthly returns of the three enhanced momentum strategies are calculated as follows:
All of them scale a standard momentum model by multiplying its monthly returns by a weight, which is either constant, focused only on downside volatility, or dynamic in that it adjusts for both forecasted returns and volatility.
All three enhanced momentum strategies outperform a standard one (Chart 1). For the US sample, which goes back to the 1930s, the dynamic volatility-scaled model performs best. It yields average monthly returns of 1.16%, compared with 0.6% in the standard model. The Sharpe ratio (SR) is also higher (0.91 vs 0.47), and the strategy has a smaller maximum drawdown.
The better performances of the enhanced strategies come from their ability to avoid momentum crashes. The dynamic strategy, which by design also accounts for lower expected momentum returns (not just higher volatility) in bear markets, is almost perfectly normally distributed.
Other factors cannot explain the superior risk-adjusted returns of these enhanced models. According to the authors, ‘the alphas of all three enhanced strategies with respect to the Fama-French factors plus MOM are economically and statistically significant’.
This means that volatility-scaled momentum models offer new and significant information, even beyond standard momentum models. For factor investors, the results imply that the three enhanced momentum models only add to the traditional three- or five-factor Fama-French models.
Lastly, on transaction costs, the authors find that all enhanced strategies are at least as implementable as a standard momentum strategy. This is because their breakeven costs, i.e., transaction costs that theoretically would render the strategies insignificant, are higher.
Do these volatility-scaled momentum models work in other countries? Yes, but not all (Appendix: Table 1).
In emerging markets, standard momentum models only provide statistically significant positive monthly returns in Chile, India, Mexico, Pakistan, Poland and South Africa. For all these, the enhanced strategies improve on that performance.
Meanwhile in Greece, Indonesia, Malaysia, South Korea, Taiwan and Thailand, where standard momentum models do not work, the volatility-scaled strategies do.
In developed markets, Japan is the only country where none of the momentum models yield statistically significant results. Meanwhile, the best performances can be found in Hong Kong and Portugal, where the enhanced strategies yielded average monthly returns of roughly 2%.
Another way of determining the effectiveness of the enhanced momentum strategies is by looking at the maximum drawdowns. The paper finds that for most countries, the maximum drawdowns are reduced for the enhanced strategies compared with MOM, providing further evidence for the lowered crash risk (Chart 2).
If the above is not helpful, the authors mention alternative methods of potentially enhancing momentum strategies.
Some early literature found large benefits from hedging a momentum strategy to a size (Small-Minus-Big) and market factor. This substantially reduces the volatility of the strategy without a loss in return.
Others have focussed on firm-specific momentum, which does not tend to experience long-term reversals. Also known as residual or idiosyncratic momentum, it has been found to exhibit only half of the volatility of standard momentum without any significant decrease in returns.
In 2021, we also covered a paper that focused on cross-sectional momentum. Here, the gap between the cumulative returns of winners and losers over the formation period was a key determinant of momentum profits.
The relative stability of pre-pandemic markets appears to be long gone – uncertainty and volatility has been a feature of 2022. This paper provides one way for investors to minimise the drawdowns in such an environment. Conceptually their approach makes sense, condition returns not only on traditional momentum, but on volatility too. Practically, the returns look promising too, especially in more developed economies.
Perhaps it is something to incorporate into your next trading strategy!
Spring sale - Prime Membership only £3 for 3 months! Get trade ideas and macro insights now
Your subscription has been successfully canceled.
Discount Applied - Your subscription has now updated with Coupon and from next payment Discount will be applied.