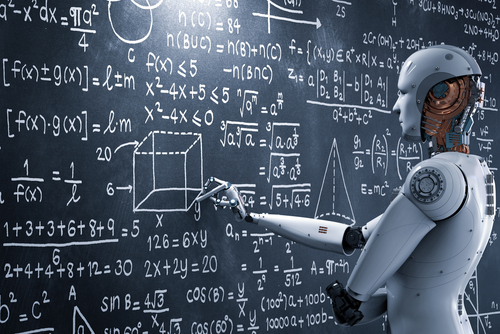
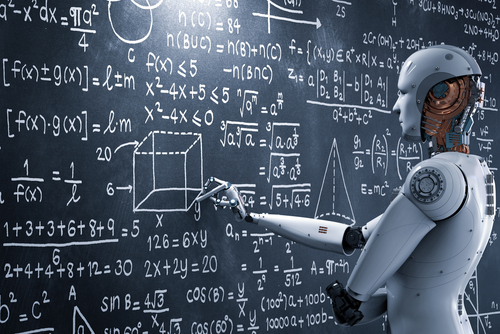
Introduction
I have been eager to test ChatGPT’s ability to summarise academic work – probably because I am wondering whether it could put me out of job. To get ahead of the curve, I thought I would see whether I could use my AI competitor as a friend before treating it as a foe. So, in this Deep Dive, I am teaming up with ChatGPT to summarise a new FEDS working paper. I ask the questions, and ChatGPT’s answers them!
This article is only available to Macro Hive subscribers. Sign-up to receive world-class macro analysis with a daily curated newsletter, podcast, original content from award-winning researchers, cross market strategy, equity insights, trade ideas, crypto flow frameworks, academic paper summaries, explanation and analysis of market-moving events, community investor chat room, and more.
Introduction
I have been eager to test ChatGPT’s ability to summarise academic work – probably because I am wondering whether it could put me out of job. To get ahead of the curve, I thought I would see whether I could use my AI competitor as a friend before treating it as a foe. So, in this Deep Dive, I am teaming up with ChatGPT to summarise a new FEDS working paper. I ask the questions, and ChatGPT’s answers them!
Summary
Q. What are the main results of the paper: “Recession Signals and Business Cycle Dynamics: Tying the Pieces Together”.
A.
- The authors develop a new framework for identifying recessions that combines multiple signals into a composite index.
- The results of the study show that the composite index is more accurate in detecting recessions than using individual signals in isolation.
- The authors also analyse the dynamics of the business cycle and find that the various signals are not equally effective at different stages of the cycle.
- The results highlight the importance of considering multiple signals and their interaction when detecting recessions and analysing business cycle dynamics.
Context
Q. Why is recession prediction important, and what variables do studies typically use to predict recessions?
A.
Recession prediction is important because it provides valuable information for decision-making by governments, businesses, and individuals. Accurate and timely recession predictions can help inform economic policy decisions, provide early warning signs for businesses to adjust their operations, and allow individuals to make more informed financial decisions.
Studies typically use a combination of macroeconomic and financial variables to predict recessions. Some commonly used variables include:
- Output gap
- Unemployment rate
- Industrial production
- Capacity utilization
- New orders for durable goods
- Interest rate spread
- Stock prices
- Credit spreads
- Housing starts
- Inflation rate
- Gross Domestic Product (GDP) growth rate
These variables are often used to build models that use statistical and machine learning methods to identify patterns and make predictions about future economic conditions. However, accurately predicting recessions is a challenging task, and there is often significant uncertainty and disagreement among experts about the state of the economy.
USP
Q. What makes the paper “Recession Signals and Business Cycle Dynamics: Tying the Pieces Together” unique?
A.
- Composite index: The authors develop a new composite index for detecting recessions by combining multiple signals, which is more accurate than using individual signals alone.
- Business cycle dynamics: The authors analyse the dynamics of the business cycle and the effectiveness of different recession signals at different stages of the cycle, which provides a more nuanced understanding of the recessionary process.
- Multiple signals: The authors consider multiple signals, rather than relying on a single indicator, which provides a more comprehensive view of the economy and reduces the potential for false alarms.
Overall, the paper offers a novel approach to detecting recessions that combines multiple signals and takes into account the dynamics of the business cycle, which makes it different from other similar papers.
Methodology
Q. How does the paper predict a recession?
A.
The paper predicts a recession using binary dependent variable regression (logit). The regression model predicts whether a recession quarter is likely to occur in the next 2, 4, or 8 quarters.
The regression equation considers all possible combinations of five predictors, which are the term spread, the Baa corporate bond spread, the change in the OECD’s composite leading indicator, the four-quarter change in the price index for personal consumption expenditures (inflation), and the unemployment rate.
The recession event is defined as an increase in the unemployment rate that exceeds the 80th percentile of the change in the unemployment rate, a change in real GDP that falls below the 20th percentile, or a recession dummy equal to 1 if the National Bureau of Economic Research identifies a recession.
The prediction equation calculates the probability of a recession event using the values of the predictor variables at a given time.
Correlations
Q. Are there correlations between the five predictors?
A.
Yes, there are correlations among the economic indicators mentioned in the text, such as inflation and the term spread being highly negatively correlated, and the term spread and the unemployment rate being highly positively correlated.
The correlations suggest that considering multiple indicators, such as inflation and unemployment, may be important for assessing the information in the term spread. The yield curve may overstate the recession signal variables if these factors are not considered at the same time, because some of the signals contained in the yield curve for recession risk stems from the yield curve’s correlations with the business cycle.
The correlations also indicate that certain patterns tend to occur one year before a recession, such as a flat yield curve, a falling leading indicator, high inflation, and/or a low unemployment rate.
(Note: ChatGPT does not provide illustrations, so I add Chart 1 to support its summary)
Recession Prediction
Q. What do the results show?
A.
The results show that the traditional predictors like the term spread, the OECD’s leading indicator, and corporate bond spread are good for recession prediction at short horizons (4 quarters ahead), whereas unemployment and inflation aid prediction at medium horizons (4 quarters four quarters ahead). The study confirms previous literature findings that prediction is poor beyond one year, but this can be improved by considering inflation and the unemployment rate.
(Note: ChatGPT lacked some interesting details here. Under the NBER’s definition of a recession, the yield curve alone is the best predictor of a recession over the next 4 quarters (Chart 2a). However, if we want to predict large decreases in real GDP over the next year, then the term spread and the OECD’s leading indicator are best (Chart 2b).)
Q. According to the paper, is there value in looking beyond the term spread for recession prediction? And why?
A.
Yes, there is value in looking beyond the term spread for recession prediction. The term spread, which is the difference between long-term and short-term interest rates, has historically been a strong predictor of recessions, but it is not the only indicator that should be considered.
Other factors such as the unemployment rate and inflation should also be taken into account when predicting recessions over the medium horizons. Inflation is in 14 of the 15 best fitting equations for recession predictions.
The unemployment rate and inflation provide a more comprehensive picture of the health of the economy and can provide valuable insights into potential economic downturns.
Additionally, the term spread may not always accurately reflect changes in the economy, and relying solely on it as a predictor can lead to false signals or missed opportunities for early detection of a potential recession.
Q. Does a failure to account for the unemployment rate and momentum overstate the role of the term spread?
A.
Yes. Momentum, as captured in the OECD’s leading indicator, is important for recession prediction at short horizons as a (positive or negative) shock is initially followed by movements of activity in the same direction. The level of the unemployment rate is important at medium horizons, as the effects of shocks fade and activity returns to its baseline value. Failure to account for these two factors overstates the role of the term spread in recession prediction.
Bottom Line
Q. Summarise the paper “Recession Signals and Business Cycle Dynamics: Tying the Pieces Together”
A.
The paper provides a comprehensive analysis of recession signals and their relationship to business cycle dynamics. The authors aim to synthesize existing research in this area and offer new insights into the relationship between various recession signals and the broader business cycle. They provide a framework for evaluating and analyzing recession signals, and examine how these signals can be used to make more informed predictions about the timing and severity of future recessions. The ultimate goal of the paper is to improve our understanding of how the economy works and to provide a more nuanced view of the economy and its potential for growth or contraction.
Q. Does the paper have any real-world implications?
A.
The paper has real-world implications as it provides a deeper understanding of the relationship between recession signals and business cycle dynamics. This understanding can be used by economists, policymakers, and investors to make more informed decisions about the economy and the potential for future recessions.
The insights provided by the authors can help individuals and organizations better anticipate and prepare for economic downturns, and support more effective decision-making related to investments, spending, and resource allocation. By improving our understanding of the business cycle and the signals that indicate a potential recession, the paper has the potential to support more stable and sustainable economic growth in the future.
My Assessment of ChatGPT
Firstly, and most importantly, I do not think ChatGPT will put me out of a job just yet. It is an excellent tool for summarising texts but, without direction, it is prone to missing key details. In many cases it needs a human to feed it the right questions, and trying to get to the more nuanced results can be a very time consuming process that leads to the human getting frozen out for asking too many questions!
That said, it is an excellent companion. ChatGPT writes exceptionally well and was very good at teasing out the broader themes in the paper. It also had a good handle on the literature and could provide context around the topic. Moving forward, I may use ChatGPT to write my summary bullet points.
Sam van de Schootbrugge is a Macro Research Analyst at Macro Hive, currently completing his PhD in international finance. He has a master’s degree in economic research from the University of Cambridge and has worked in research roles for over 3 years in both the public and private sector.