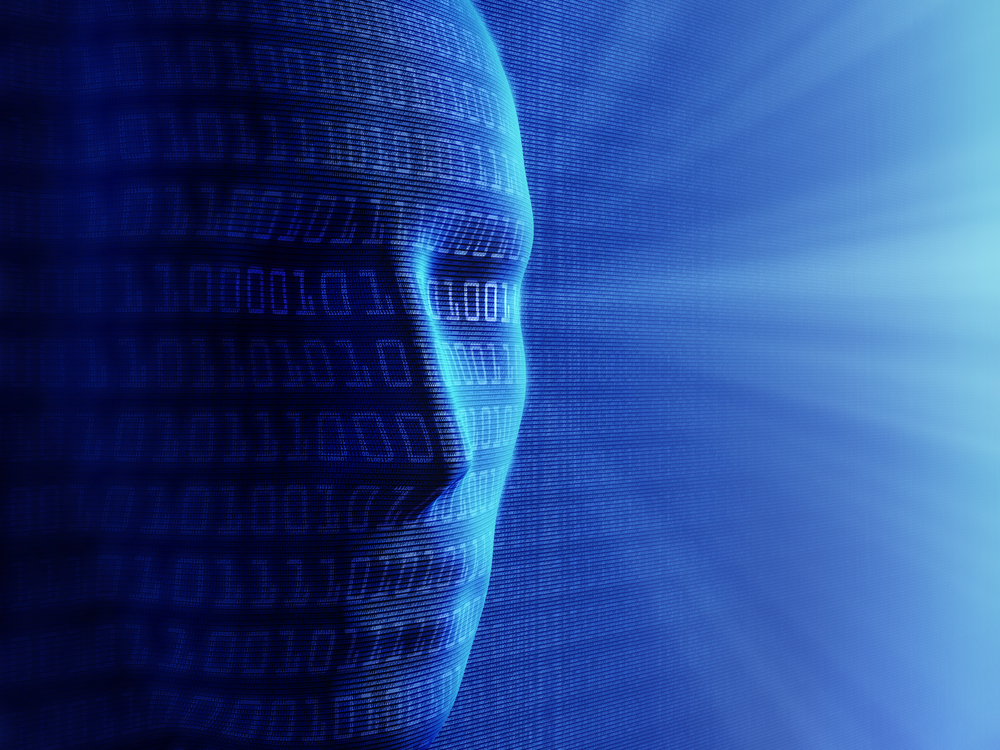
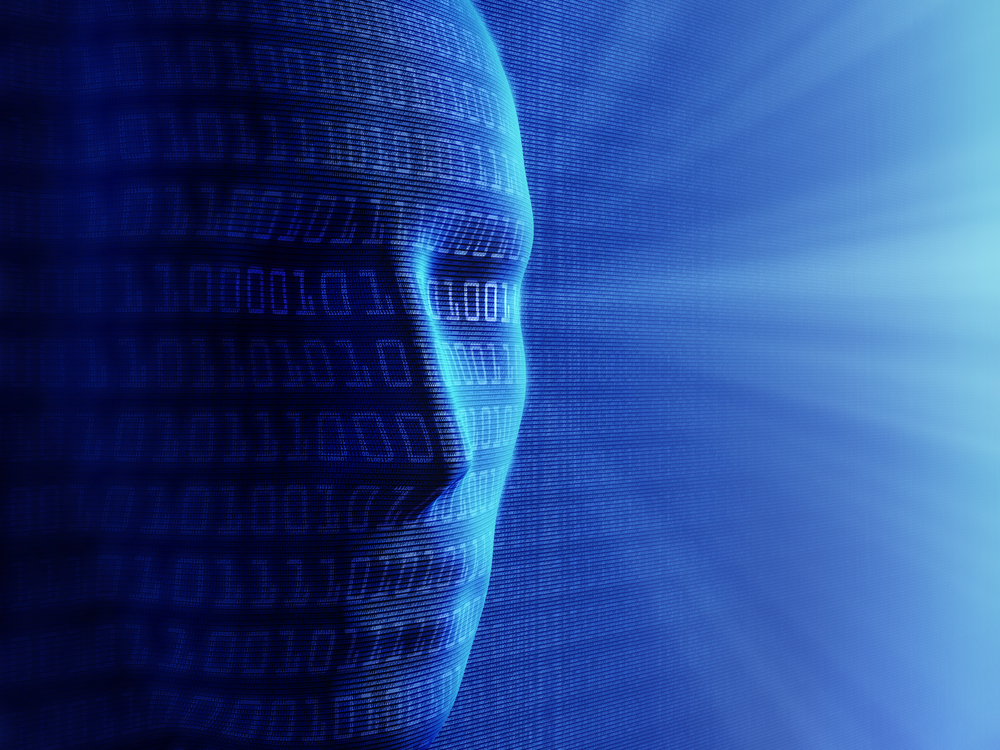
AI is all anyone can talk about since the advent of ChatGPT. From revolutionizing healthcare with personalized medicine to generating trading signals in financial markets, every industry is claiming a new future for itself thanks to the technology. And perhaps rightly so.
But how smart is this new technology? And perhaps more fundamentally, how do we even test it? As we recently explored in a podcast with Professor Melanie Mitchell, AI is running into major roadblocks outside strictly defined tasks. And we are struggling to work out how to improve it.
First Impressions? AI OK
The realm of AI is advancing at a breakneck pace. One of the most thrilling advances is in the domain of natural language processing (NLP), epitomized by models like OpenAI’s GPT-3. This behemoth of technology, wielding a whopping 175 billion parameters, can draft essays, solve coding problems, and even compose poetry with a finesse that blurs the lines between human and machine authorship.
The impact? A revolutionary shift in how we interact with digital systems, making technology not just a tool, but a collaborative partner that understands and responds with human-like nuance.
And it’s not just the spectators who are impressed. Even those inside the AI industry were shocked at the advances. For Melanie Mitchell, it all began with speech recognition:
‘You started to get machines that could transcribe speech to text. You could tell your phone, you could dictate an email to your phone, and it would do a very good job of transcribing it. The way that that worked was not by giving the machine any special knowledge of grammar or how speech maps onto grammar. It wasn’t engineered at all. It was just machine learning, basically training systems on vast amounts of speech data to do this task of transcribing it to text. So a statistical machine learning model was able to capture this, and people, including, me were very surprised.’
And with large language models trained on exponentially more data than these early speech recognition tools, we went into overdrive. The more we scaled the compute power, the better they seemed to become. Now, you get models that can pass the legal bar, do an MBA, or solve complex mathematical equations.
But Is Passing the Legal Bar Enough to Pass the Intelligence Bar?
Just as we are now starting to realize that giving humans standardized tests is a problematic method for testing intelligence, so it is for AI.
First, there’s the mystery of the models’ training data – specifically, whether the test questions have been previously seen during their extensive training on vast internet datasets, a critical detail companies selling these models typically refuse to disclose. As Melanie Mitchell puts it, ‘You don’t want to test on the training data. That’s like number one rule in learning.’
Second, there is the nature of the models’ problem-solving strategies, which might differ fundamentally from human methods. They may rely on detecting statistical patterns that are beyond human perception, casting doubt on how these capabilities might translate to real-world scenarios.
This makes it critical to test not just whether the machine passes or fails, but the robustness and distribution of its answers across multiple variables. This approach helps identify weaknesses in the AI’s understanding and response mechanisms, safeguarding against errors when faced with unexpected inputs or in diverse contexts.
The AI Horse Needs Its Human Whisperer
One of the main problems with AI is their sensitivity to prompts – and their dependence on them to produce half-decent answers. Prompting finetunes the interaction, steering the technology to generate more accurate and relevant responses. It transforms vague questions into targeted inquiries, often helping the AI follow a logical path to an answer.
But worryingly, it is a vague field. As Melanie Mitchell puts it,
‘People have likened it to alchemy because we don’t know why certain incantations in the prompts make the machine work. There’s been papers where people show, well, if you say take a deep breath in your prompt, it’ll improve the performance. And it’s not a science yet, but it does show that the machines are brittle. They are not robust. If I talk to you, I don’t have to engineer very much what I’m saying. I think I don’t have to think very hard. I know that you understand. Whereas with a machine, you have to think pretty hard about how you’re going to present a problem to it.’
Conclusion? Your Job Is (Probably) Still Safe
If you work outside AI, you are in for a comforting conclusion. We are likely to end up with a hybrid approach of human plus machine for most tasks. According to Melanie Mitchell, these machines lack two key things.
The first is episodic memory. As Melanie puts it, ‘You can remember your past, and you have abstracted a lot of that memory so that you remember it in a more abstract way, you might not remember it exactly. But when something new happens that reminds you of something that you’ve experienced in the past, then that helps you figure out what to do.’ The machines lack that.
The second are the internal models of the world we walk around with. Humans excel at creating and manipulating mental models, such as visualizing and rearranging stacked boxes. In contrast, the ability of language models to perform similar tasks is limited and controversial. These AI systems attempt to track conversational context but often do so in a brittle and less intuitive manner compared to human cognition.
So keep your chin up, you’ll likely still have a use even as AI becomes part and parcel of everyday work experience. Just make sure you know how to whisper to it…
Matthew Tibble is Commissioning Editor at Macro Hive. He has worked as an editorial consultant and freelance editor for companies such as RiskThinking.AI, JDI Research, and FutureScape248.