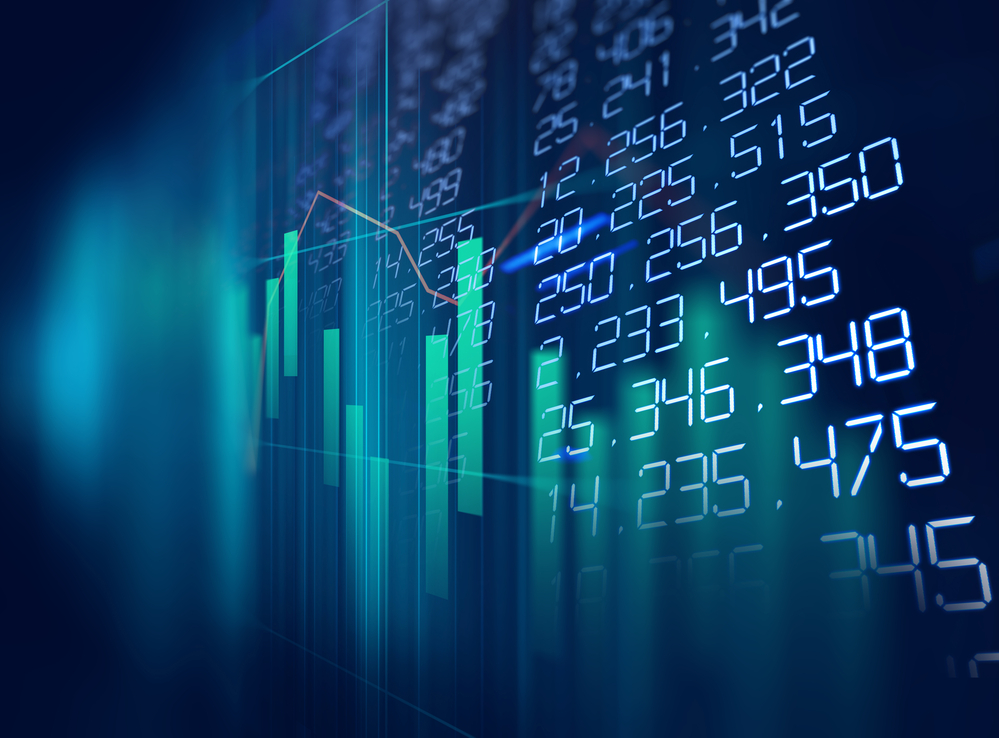
AI | Monetary Policy & Inflation
AI | Monetary Policy & Inflation
This article is only available to Macro Hive subscribers. Sign-up to receive world-class macro analysis with a daily curated newsletter, podcast, original content from award-winning researchers, cross market strategy, equity insights, trade ideas, crypto flow frameworks, academic paper summaries, explanation and analysis of market-moving events, community investor chat room, and more.
Not a day goes by without a new LLM model hitting the market, each claiming to outperform the rest on some benchmark leaderboard. But these self-reported scores often deserve scepticism. Much like marking your own homework, these evaluations can be misleading. For starters, many benchmark datasets – or even their answers – might appear in a model’s training data. And then there’s the risk of ‘benchmark gaming,’ where model builders torture prompts or sampling settings until they get the results they want.
The real test? Evaluating models on genuinely out-of-sample, real-world tasks.
At Macro Hive, we are in a unique position to do just that—thanks to our proprietary, paragraph-level labelled dataset of central bank communications. Using this dataset, we benchmarked a range of top LLMs: Claude 3.7, DeepSeek R1, OpenAI’s GPT-4.5, and our own fine-tuned GPT-4o-mini variant.
The results: the Macro Hive fine-tuned GPT-4o-mini model achieved 88% accuracy, outperforming every other model. GPT-4.5 followed at 78%, with DeepSeek R1 at 76% and Claude 3.7 Sonnet (Extended) at 75%.
In financial markets, traders often interpret central bank statements, minutes, and speeches to infer policy stance – classifying them as dovish (accommodative), neutral, or hawkish (tightening). Traditionally, economists do this work. But LLMs are now capable of interpreting such nuanced language too.
At Macro Hive, we have fine-tuned several LLMs to take on this task, using GPT-4o-mini as our base model. The cornerstone of our approach is our unique labelled dataset, built by our economists at both paragraph and sentence levels – granularity that is rare in the field. Crucially, we continuously expand this dataset, allowing us to test models on a truly out-of-sample basis.
Each model was tested in a standardised zero-shot setup, using a single prompt with no examples, to provide a clean comparison of their raw capabilities.
Our fine-tuned GPT-4o-mini ranked #1 with 88% accuracy. That is impressive, especially given that GPT-4o-mini is not OpenAI’s top-tier model. It shows how domain-specific fine-tuning can beat larger, more expensive alternatives.
Second place: OpenAI’s largest model, GPT-4.5, at 78% accuracy.
Third and fourth: DeepSeek R1 (76%) and Claude 3.7 Sonnet (Extended) (75%).
But here is the kicker: price. GPT-4.5 costs ~$75 per million tokens, Claude 3.7 Sonnet costs ~$3 per million tokens, DeepSeek-R1 costs ~$0.55 per million tokens, while our fine-tuned GPT-4o-mini costs just ~$0.30 per million tokens.
Key Performance Highlights:
Breaking down performance by class reveals each model’s strengths or biases in detecting specific tones. The top-performing model, Macro Hive Fine-Tuned GPT-4o-mini, shows a strong balance — correctly identifying 85% of dovish, 86% of neutral, and 91% of hawkish paragraphs, leading in all three categories. OpenAI’s GPT-4.5 also performs consistently (81% dovish, 76% neutral, 77% hawkish), albeit at a lower level than our fine-tuned model.
Key Performance Highlights:
These results show that carefully labelled domain-specific datasets – combined with thoughtful fine-tuning – can outperform much larger, more expensive models.
Macro Hive’s fine-tuned GPT-4o-mini ranks highest with 88% accuracy and a cost of just ~$0.30 per million tokens, far ahead of GPT-4.5’s 78% at ~$75.
Moreover, fine-tuning does not just boost accuracy – it also improves class balance and removes biases seen in general-purpose models. This makes fine-tuned smaller models the smart, scalable, and cost-effective choice for real-world tasks.
Spring sale - Prime Membership only £3 for 3 months! Get trade ideas and macro insights now
Your subscription has been successfully canceled.
Discount Applied - Your subscription has now updated with Coupon and from next payment Discount will be applied.