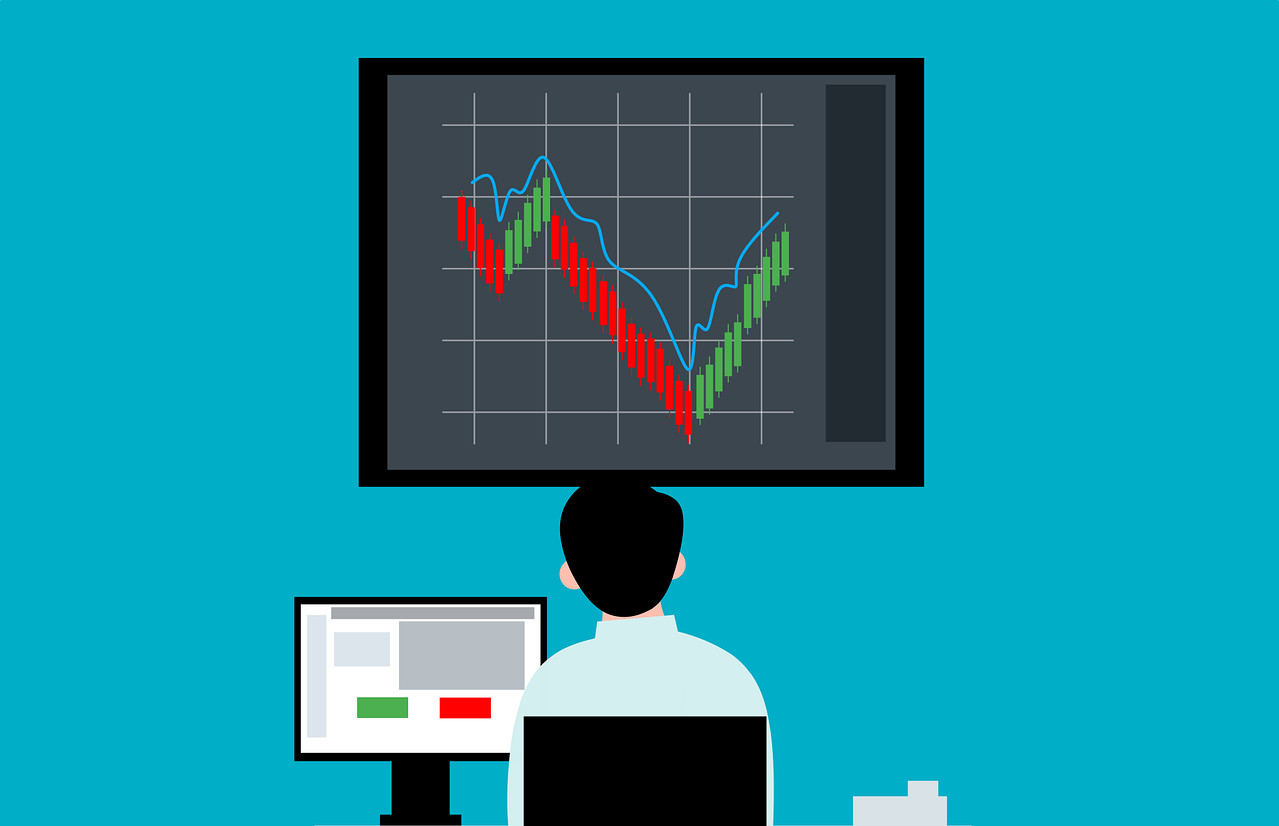
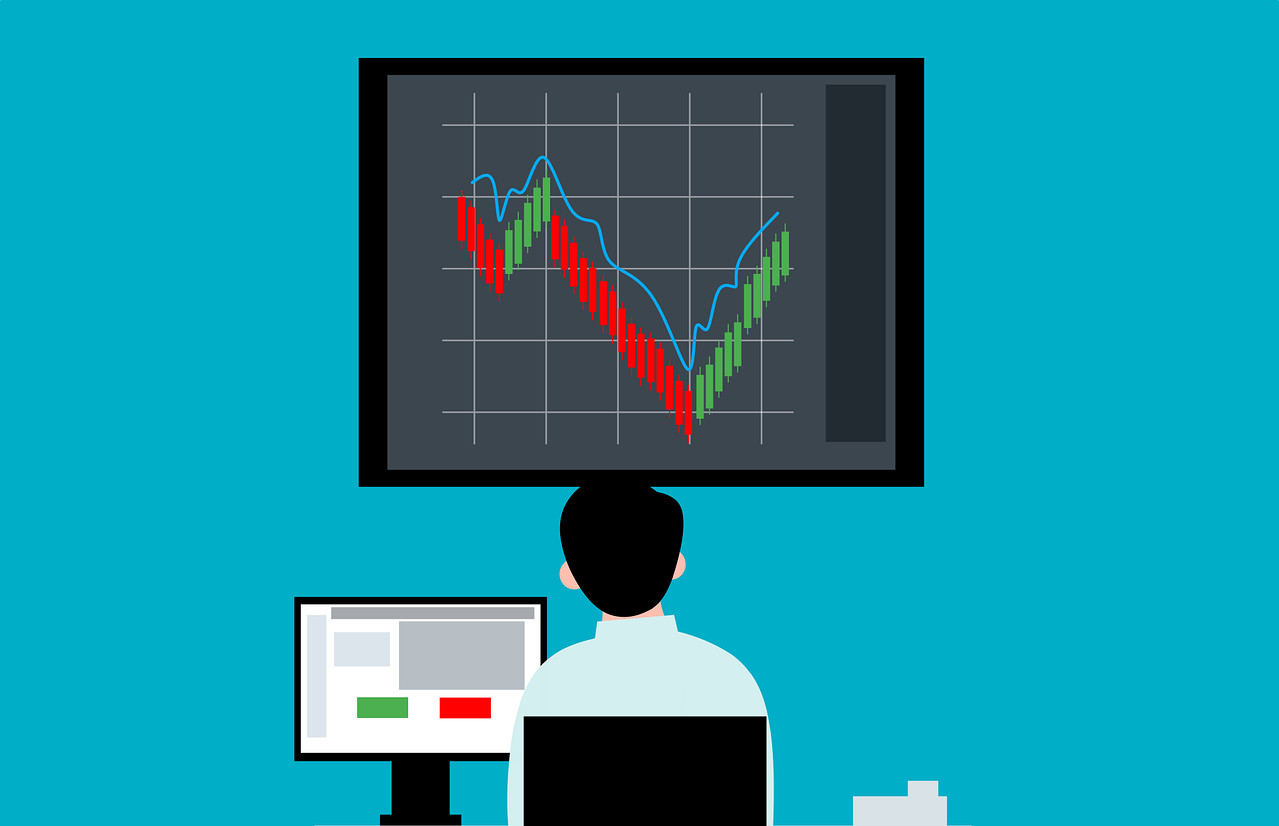
Summary
- A new NBER working paper examines which models are best for forecasting the nominal exchange rate.
- It finds evidence that the S&P 500 can help when forecasting the nominal exchange rate over one-, 12-, 36- and 60-month horizons.
- However, global risk factors generally add little additional forecasting power when the level of the nominal exchange rate is included as a predictor.
- For the models to outperform a random walk, stationarity must be assumed. In larger sample simulations, there is little conclusive evidence that models can forecast nominal exchange rates.
Introduction
Outperforming a random walk model when trying to forecast nominal exchange rate movements is notoriously difficult. Meese and Rogoff (1983) famously admitted that, while theories suggest the exchange rate is determined by key macroeconomic fundamentals, forecasting the exchange rate to remain unchanged was a better prediction than using various fundamentals-based models.
In recent years, however, studies have shown that global risk factors could help when forecasting the dollar. A new NBER explains why this may not be the case, and under which conditions using the level of the log of the nominal exchange rate is actually better. Specifically, they find the following:
- Including the nominal exchange rate as a predictor in a forecasting model of future changes in the nominal exchange rate improves its performance relative to a random walk.
- Over a one-month horizon, there is evidence that the detrended S&P 500 level, the Repo and the 10-year to two-year US term spread are helpful in forecasting the nominal exchange rate.
- A bivariate model that includes the nominal and real exchange rates as predictors is perhaps the best model for predicting the nominal exchange rate over 36- and 60-month horizons.
- The above results hold only when assuming the nominal exchange rate is stationary. Otherwise, the models are not generally better than a random walk, except for EUR/USD movements.
Difficulties in Forecasting the Nominal Exchange Rate
A variable is said to be stationary when its mean and variance are time invariant. Stationarity is crucial in avoiding spurious correlations in time series analysis. A non-stationary variable, on the other hand, makes mistaken inferences easy when assessing forecasts, and a variable that contains a unit root is non-stationary. A unit root process has a mean that is either growing or decreasing over time, and any shocks that affect a unit root variable will have a persistent impact on its mean.
The USD relative to the other G10 currencies appears to be a borderline stationary random variable, but it is very persistent. If dollar exchange rates are stationary, then forecasting it should be relatively easy. One key issue, however, is that its persistence may reflect the presence of a unit root, which can cause problems in statistical inference. Indeed, if exchange rates contain a unit root, it may render them entirely unpredictable, explaining why models find it difficult to outperform random walk models.
Source: Paper, page 57
Methodology
The authors look at the statistical inference from in-sample and out-of-sample nominal exchange rate forecasts over one-, 12-, 36-, and 60-month horizons. They start with the assumption that exchange rates are stationary and assess the forecasting performance when using (i) the level of the log exchange rate, (ii) global risk measures, (iii) a combination of (i) and (ii), and (iv) the level of the log of the real exchange rate. The authors assess their performance against each other and against a random walk process.
The decision to include global risk measures derives from the premise that the dollar strengthens during periods of global distress. In all, twelve macro variables are included:
- The US Treasury premium – one-year covered interest parity deviation of government yield between the US and a foreign country.
- MAR global factor – constructed by Miranda-Agrippino and Rey (2020); this is extracted from a dynamic factor model of a wide range of world asset price series.
- The GZ spread – an unweighted cross-sectional average of US corporate nonfinancial credit spreads.
- A linearly detrended log of the S&P 500.
- The log of the VIX Index.
- A term spread (5y-FF) – difference between five-year US Treasury yield and overnight Fed Fund rate.
- A term spread (10y-2y) – difference between 10-year US Treasury and two-year US Treasury.
- TED – yield difference between US dollar LIBOR rate and US Treasury at a three-month horizon.
- Intermediary capital ratio – market capitalization-weighted average of New York Fed primary dealers’ equity to asset ratio.
- Intermediary weighted return – market capitalization-weighted equity return of the holding companies of the primary dealer of New York Fed.
- Repo – linearly detrended log of Overnight Repo outstanding of the primary dealers.
- Commercial Paper – the linearly detrended log of Financial Commercial Paper outstanding of the primary dealers.
If Exchange Rates Are Stationary…
Within sample, the level of the log exchange rate exhibits very good forecasting power over 12-, 36-, and 60-month horizons. A one unit rise in the current level of the log exchange rate forecasts a mean reversion in the future level of the exchange rate over the next 12 months (Chart 2). That mean reversion is stronger for USD/SEK and increases the larger the forecasting horizon.
However, out-of-sample performance is generally considered more important when evaluating forecast performance. On this front, a forecasting model that includes the level of the log exchange rate produces better predictions than a random walk model. This is true for all cross-currency dollar pairs.
The level of the log exchange rate is also better at forecasting the USD than all 12 global risk factors over the medium term. Interestingly, however, the S&P 500 and repo have better predictive power over a one-month horizon. In fact, the S&P 500 generally has very good or excellent forecasting power across all horizons (Table 1). And over a 12-month horizon, it is significant for predicting movements in all nine currencies. Importantly, using any of the 12 variables improves the forecasting performance relative to a random walk.
Better still, according to the out-of-sample estimates, including the level of exchange rate improves forecasts further. In other words, a bivariate model with a global risk factor and the exchange rate is better than a univariate model with only a global risk factor. However, only a bivariate model with 5y-FF term spread and the exchange rate is consistently better (over 12-, 36- and 60-month horizons) than a univariate model with only the exchange rate.
Lastly, the authors include the log of the real exchange rate as a forecasting variable. The results show that this is helpful in forecasting future changes in the nominal exchange rate. The real exchange rate has significant forecasting efficacy at the 12-, 36- and 60-month horizons. Like global risk factors, although the real exchange rate has predictive power, it is never better at producing forecasts than the level of the log nominal exchange rate. Meanwhile, a model which includes both performs best.
In sum, all models – whether they include only the level of the log nominal exchange rate, a global risk factor and/or the real exchange rate – outperform a random walk at forecasting future changes in the nominal exchange rate. The best model over the longer-term horizon (36-60 months) is one that includes as independent variables the nominal and real exchange rates. Over a one-month horizon, it is one that includes the S&P 500 and nominal exchange rates, while over a 12-month horizon the best model includes 5y-FF (or TED) and nominal exchange rates.
Does Small-Sample Bias Affect the Results?
Economic theory points towards the nominal exchange rate incorporating a unit root. This is because the real exchange rate (RER) is generally considered stationary, and so changes in either the nominal exchange rate or price deviations must drive short-term deviations in the RER. In economies where policymakers use interest rates to respond to inflation rates, nominal exchange rates move most as they adjust to the price level differential between two countries.
As mentioned earlier, the empirical evidence suggests that the log dollar exchange rates are borderline stationary, at least during the 21st century. However, small-sample biases make forecasting the dollar at medium- to long-term horizons susceptible to mistaken inferences. As such, the authors run the same models using large sample simulations to overcome the small-sample biases that in the previous section could have led us to conclude incorrectly that we can forecast the dollar exchange rates.
Under this scenario, the authors find no strong evidence against the random walk model from the within-sample analysis. Out-of-sample forecasts show that only the USD/EUR rate would reject the null of the random walk at a 5% level. The authors conclude, therefore, that other than for the USD/EUR exchange rate, there is little evidence that the level of the exchange rate or global risk factors can help forecast future exchange rate changes.
Bottom Line
The results suggest that, when forecasting future nominal exchange rate changes, the level of the log of the exchange rate is the single most important predictor. Researchers may wish to include global risk factors, but there is only limited additional forecasting power granted from doing so. Over the long-term horizon, adding the real exchange rate as a predictor appears to have much value.
Citation
Engel C., Pak Yeung Wu S., (2021), Forecasting the US Dollar in the 21st Century, NBER, (28447), https://www.nber.org/papers/w28447
Sam van de Schootbrugge is a macro research economist taking a one year industrial break from his Ph.D. in Economics. He has 2 years of experience working in government and has an MPhil degree in Economic Research from the University of Cambridge. His research expertise are in international finance, macroeconomics and fiscal policy.
(The commentary contained in the above article does not constitute an offer or a solicitation, or a recommendation to implement or liquidate an investment or to carry out any other transaction. It should not be used as a basis for any investment decision or other decision. Any investment decision should be based on appropriate professional advice specific to your needs.)