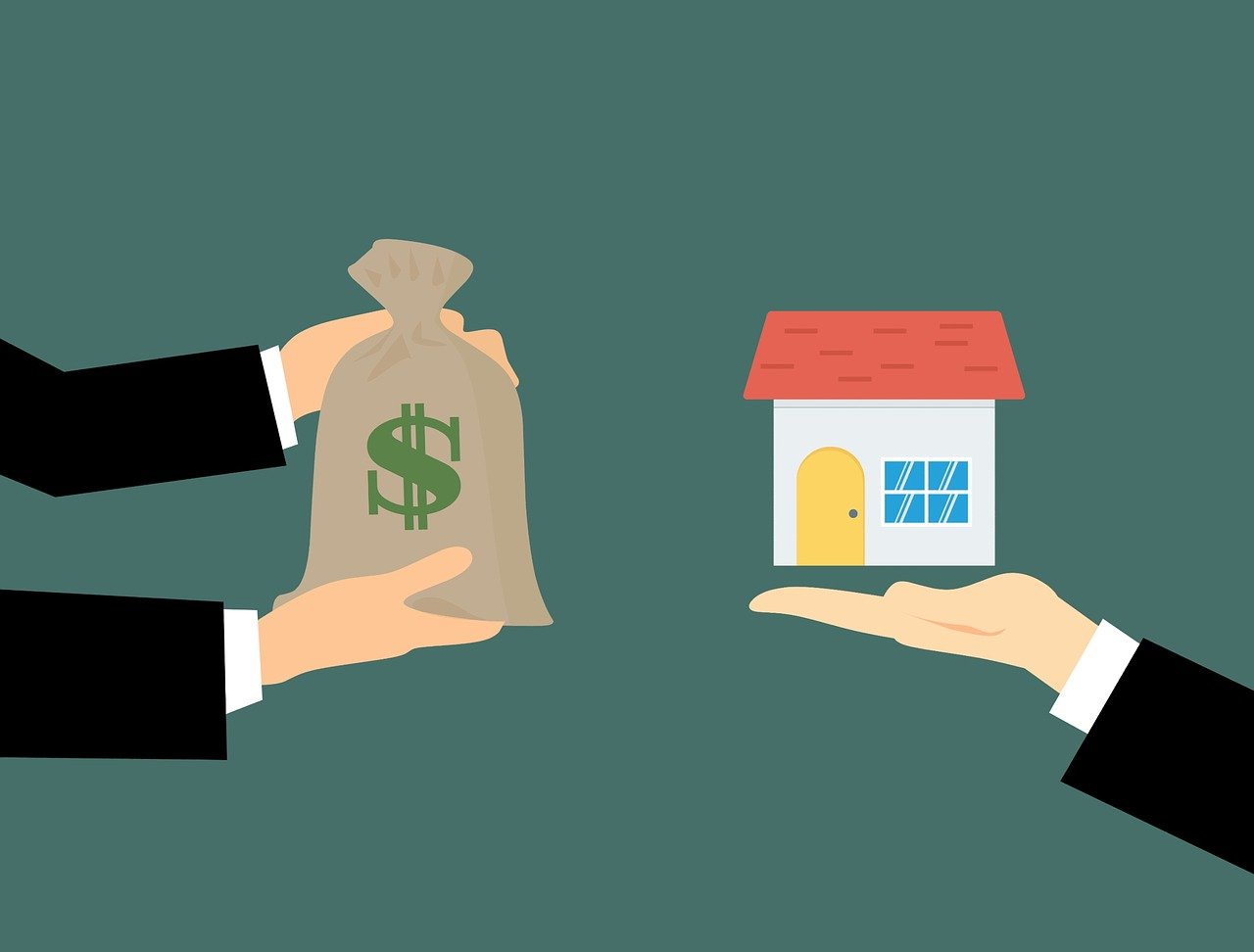
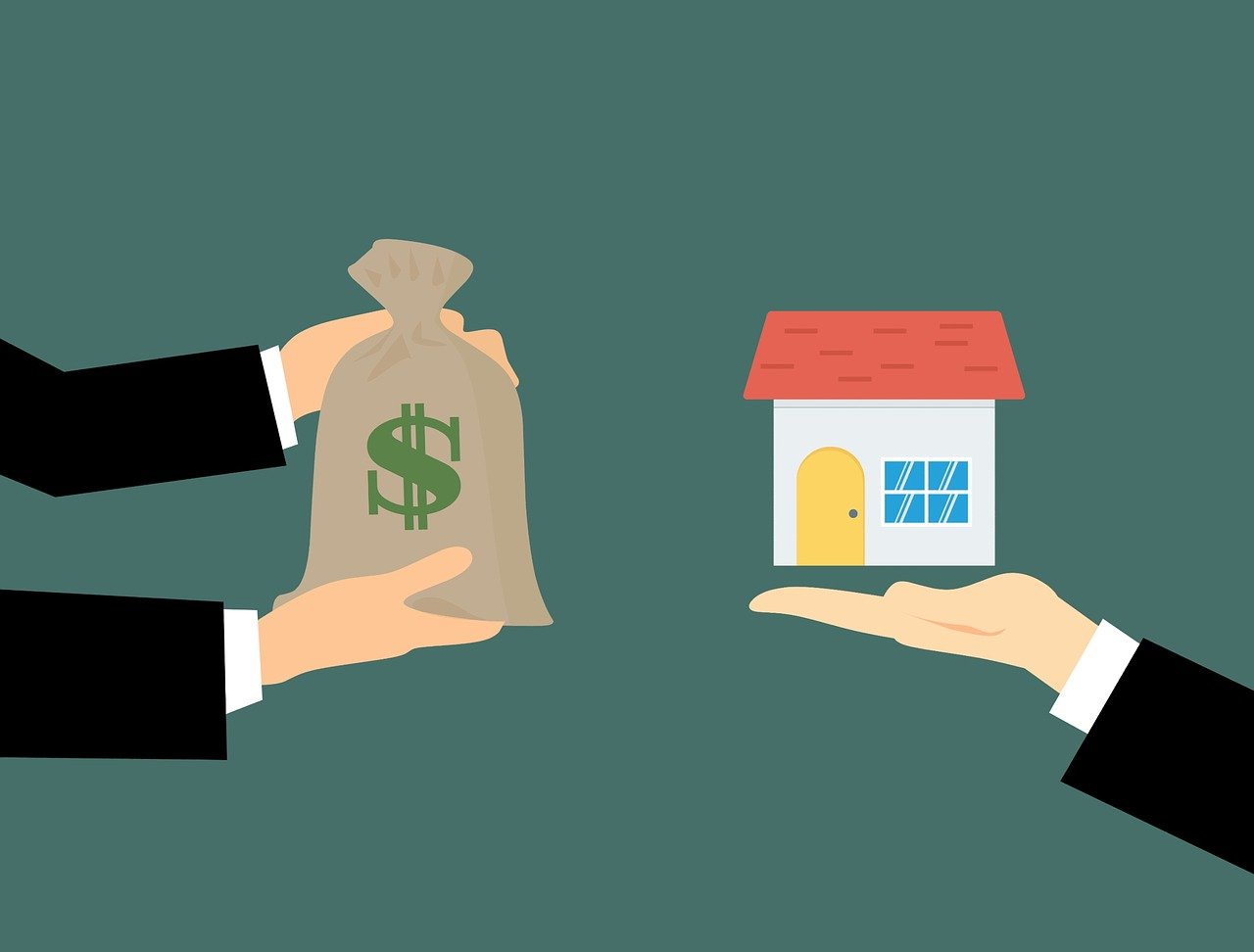
Summary
- One of big data’s many benefits is that it can supplement lower-frequency macroeconomic data with daily series across a wide range of financial predictors.
- A new Swiss Finance Institute research paper explores whether financial market data can help predict US house prices.
- Returns in the S&P 500, REITs, and construction and finance industries all predict residential housing market prices well.
Introduction
We now generate more data in two days than we did from the dawn of time until 2003. The finance profession is harnessing that information in increasingly innovative ways. A new Swiss Finance Institute research paper analyses over 70 mixed-frequency macroeconomic and financial variables to assess their ability to backcast, nowcast and forecast US house prices. Specifically, they ask whether investors can better predict today’s residential housing market returns than the two-month-lagged Case-Shiller index. They find:
- Financial variables improve house price predictability, especially at higher frequencies. The best performers are the S&P 500, REITs, and the construction industry portfolio returns.
- Relative to financial predictors, macroeconomic variables offer limited improvement, with housing permits a notable exception.
- During periods of economic stress and market volatility, financial predictors offer a far better indication of current house prices than the Case-Shiller index.
The ‘Big Data Revolution’
A wave of new research is pushing the boundaries of big data analysis in finance. Researchers showed that ML can outperform the actual selection of new board members, currently done by humans. One paper quantified corporate culture to understand its implications across firms. Some explored whether machine-based trading affects the efficacy of market microstructure measures developed before machines came to dominate trading volume.
Others identified agency conflicts between institutional traders and their brokers using a particularly large dataset. Researchers even used webpage clickstream data from Bitly to understand the role of uncertainty in financial trading. And it was shown how ML can perform multiple hypothesis testing in linear asset pricing models, focusing on addressing data snooping. The following Swiss Financial Institute research paper shows how big data can also improve forecasts of house prices.
Predicting House Prices
Most real estate indices, including the industry-standard Case-Shiller (CS) index, are released with several months’ delay. Therefore, home price valuations made today using the CS repeated-sales index from two months ago would potentially miss relevant information. The authors of a Swiss Finance Institute research paper examine what information can be elicited from other macroeconomic and financial variables to help improve today’s house price valuations.
The question they ask is straightforward. Assume the current month is March and the latest CS data is available for January. Can they predict house prices in February (backcasting), March (nowcasting), April and June (forecasting) better with additional macroeconomic and financial variables than just using past CS values? In essence, the benchmark is a simple autoregressive model using past CS values, which they pitch against a forecasting model that includes an additional 71 macroeconomic and financial indicators.
Data, Variables and Methodology
The authors collect data from 1987 to 2019 on seasonally adjusted S&P/Case-Shiller HPI, 38 financial variables and 33 widely used macroeconomic indicators (Appendix). Financial variables include the value- and equally weighted S&P 500 returns; the spread between the 30-year fixed-rate mortgage and the 30-year Treasury rate; the spread between the 10-year and three-month Treasury yield; returns to aggregate, mortgage, residential, equity, and hybrid REITs; and the credit spread. Data is available at daily and monthly frequencies.
The macroeconomic variables are available at a monthly frequency and include the Chicago Fed National Activity Index; the unemployment rate; inflation rate; non-farm payrolls; new privately owned housing units started and permits; total industrial production along with twelve disaggregated indexes; the ISM Report on Manufacturing Business Employment; and a variety of average weekly hours measures. For all these predictors, they use monthly growth rates.
Their empirical strategy has four dimensions. First, they assess which of the 71 individual variables can improve real estate price predictability. Second, they determine whether grouping individual predictors can yield even better results. Third, they use MIDAS (a mixed data sampling method) to examine whether higher-frequency, daily financial data can improve forecasting performance. Finally, they see whether their results hold across 19 US metropolitan statistical areas (MSA).
The authors estimate all models (180 different specifications) using OLS, both in-sample and out-of-sample. Variables contain at most three lags to avoid the full ‘kitchen-sink’ approach. They measure the performance of each specification by the mean square forecasting error (MSFE) relative to the benchmark autoregressive model. They break the performance down into backcasts, nowcasts and forecasts.
Results
Starting with backcasting (that is predicting real estate valuations from last month), S&P 500 returns, equity, aggregate and hybrid REITs returns, and most industry returns lead to significant improvements in performance relative to the benchmark. Furthermore, all financial variables provide statistically valuable information for predicting today’s valuations (nowcasting). And, for forecasting one- and three-month(s) ahead, financial variables improve prediction accuracy by 3-6.4%.
Overall, of the 114 distinct specifications the authors consider, 35 (31%) are significant at backcasting, 70 (61%) at nowcasting, 72 (63%) at forecasting one period ahead, and 56 (49%) at the three-month horizon. Across the predictors, including one-month and two-month lags produces the largest increase in forecasting precision (Chart 1). For the macroeconomic variables, only housing permits, industrial production of residential utilities and manufacturing improve house price predictability. The results also hold at a local level and provide the best forecast for returns in Seattle.
Most financial predictors improve forecasting accuracy while macroeconomic predictors do not. So, it is unsurprising that grouping variables in this way leads to superior predicting performance by financial variables. When grouping, the authors recommend using a weighting scheme based on the inverse MSFE average.
Next are the results from MIDAS. Using higher-frequency (daily) financial data significantly improves nowcasting and forecasting performance – in most cases by at least 5%. Among the most robust MIDAS predictors are the returns of the S&P 500, the Ziman real estate indices, and various industries such as construction and finance. These sectors are directly or indirectly related to the residential real estate market.
The authors find that predictors do particularly well in turbulent times – when they are most needed. They also find that the forecasting accuracy of a model which includes the above financial predictors improves relative to the benchmark when economic uncertainty (measured by NBER recessions, VIX and UoM Consumer Sentiment Index) increases. For example, a $1mn property has an annual forecast error of around $33,300, which improves by $1,064 in normal months but $3,784 in crisis months.
Bottom Line
The authors show that a big data approach which exploits financial variables together with mixed-data sampling adds great value to forecasting residential real estate returns. Naturally, these forecasts do not necessarily equate to the realised house price valuations once the information becomes available two months later. However, including financial variables can improve forecasts relative to a model that only uses historical Case-Shiller data, in particular during periods of economic stress.
Citation
Garzoli, M., Et Al., (2021), Backcasting, Nowcasting, and Forecasting Residential Repeat-Sales Returns: Big Data meets Mixed Frequency, SSRN, Research Paper No. 21-21, https://papers.ssrn.com/sol3/papers.cfm?abstract_id=3798356
Goldstein I., Et Al., (2021), Big Data in Finance, NBER, Working Paper (28615), https://www.nber.org/papers/w28615
Appendix
Sam van de Schootbrugge is a macro research economist taking a one year industrial break from his Ph.D. in Economics. He has 2 years of experience working in government and has an MPhil degree in Economic Research from the University of Cambridge. His research expertise are in international finance, macroeconomics and fiscal policy.
(The commentary contained in the above article does not constitute an offer or a solicitation, or a recommendation to implement or liquidate an investment or to carry out any other transaction. It should not be used as a basis for any investment decision or other decision. Any investment decision should be based on appropriate professional advice specific to your needs.)