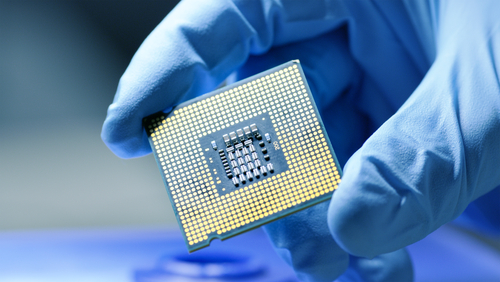
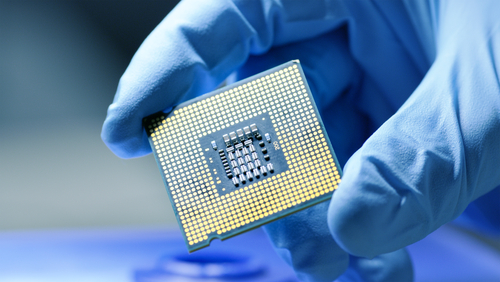
Everyone has a take on DeepSeek. In most cases, it simply re-affirms their prior views (US is weak and China is winning; US is strong, and China is cheating, etc.). But here are three things that most people are missing (well, two things really):
1). Lower Costs
DeepSeek has delivered way lower inference costs for the same results as existing models (such as ChatGPT). Moreover, they have been very open about how they built the models and have been generous with licences. Others will therefore copy them and push costs lower. All that is great for users. The big question is what this means for the tech sector. The message from markets is that AI infrastructure plays will be hit (NVIDIA, Broadcom, Oracle all down, Chart 1).
2). More Fun
DeepSeek is more unconstrained and hence more fun in its responses. It is a bit like ChatGPT in its early days, when you could ask it how would you wipe the energy grid of a country and so on. A recent paper tested how safe DeepSeek R1 was compared to o3-mini, and the answer was ‘highly unsafe.’ Basically, DeepSeek will give answers to questions that you are not supposed to ask.
A more subtle point is it is probably a good thing we have language models with different safety standards. After all, US cultural sensitivities cannot be the only way to dictate standards.
3). More Synthetic Data = More Training
On a geekier point, the focus has been on how DeepSeek has lowered inference costs, which in turn means less demand for compute. However, there are also implications for training language models. This comes down to DeepSeek being a reasoning model that uses chain of thought (CoT), which forces the model to think step-by-step. You can see it in action when you use DeepSeek.
These ‘chain of thought’ sentences can themselves be used to expand the dataset to train new base language models. Put another way, most base language models have been trained on the entire internet, so what is the new data frontier? Synthetic data! And what is CoT sentences? Synthetic data! Therefore, DeepSeek and other reasoning models that use CoT could turbocharge the generation of new (synthetic) data that spur more training of new language models. This could mean those powerful chips are needed after all.