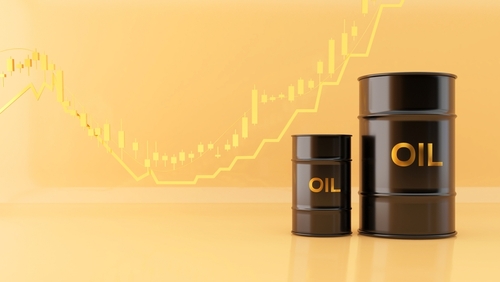
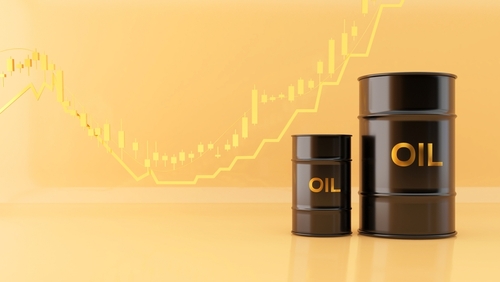
Summary
- A new Journal of Banking and Finance paper constructs a momentum model based on stock market returns and oil-implied volatility.
- The use of oil-implied volatility, or oil price uncertainty, builds on the fact that oil is a key determinant of global growth and stock prices.
- The paper’s model performs significantly better than a model that only captures momentum in stock prices.
- When applied to the S&P500, the same model significantly outperforms a simple buy-and-hold strategy and is signalling a ‘buy’ for November.
This article is only available to Macro Hive subscribers. Sign-up to receive world-class macro analysis with a daily curated newsletter, podcast, original content from award-winning researchers, cross market strategy, equity insights, trade ideas, crypto flow frameworks, academic paper summaries, explanation and analysis of market-moving events, community investor chat room, and more.
Summary
- A new Journal of Banking and Finance paper constructs a momentum model based on stock market returns and oil-implied volatility.
- The use of oil-implied volatility, or oil price uncertainty, builds on the fact that oil is a key determinant of global growth and stock prices.
- The paper’s model performs significantly better than a model that only captures momentum in stock prices.
- When applied to the S&P500, the same model significantly outperforms a simple buy-and-hold strategy and is signalling a ‘buy’ for November.
Introduction
Momentum strategies are popular. They are so popular that the first paper to systematically document their profitability is cited over 13,000 times on Google Scholar. This places it in the top 100 papers of all time, across all fields.
Their profitability has made both cross-sectional and time series momentum strategies some of the most common types of trading models. A new Journal of Banking and Finance paper focuses on the latter, augmenting a simple stock return time-series momentum model with crude oil-implied volatility (OVX).
Their strategy goes long in stocks when the previous month’s stock returns were positive and oil price uncertainty fell. It goes short when the oppositive holds. When both move in the same direction, investors hold a risk-free asset.
Between 2007 and 2021, the annual returns from this trading rule are double those of a simple buy-and-hold strategy and 1.5x higher than a traditional time-series momentum model. It also delivers a monthly Sharpe Ratio of 0.175.
Option-Implied Oil Price Volatility
Since oil’s influence on the global economy is unavoidable, oil price volatility should have predictive power over stock prices. According to the authors, one channel through which this may play out is the funding constraints of financial intermediaries. Greater oil-implied volatility increases oil derivatives’ market margins. This then increases financial intermediaries’ capital constraints, thereby reducing investment activity.
If true, a momentum model that conditions its signals on developments in the oil market should outperform one that only looks at stock returns. This is what the authors test, using changes in the Cboe Crude Oil Volatility Index (OVX) alongside changes in excess stock market returns.
Data and Methodology
The OVX data is an estimate of the expected 30-day volatility of crude oil, as priced by the US Oil Fund (USO). It is available from May 2007 and collected through to August 2021.
For the stock returns, the authors obtain monthly MSCI total return index data for various countries from Rifinitiv Datastream. They examine 59 countries. 44 are oil-importing and 15 oil-exporting. For consistency, all equity indices are collected in USD.
All returns are calculated in excess of a risk-free rate. This is the US short-term, one-month interest rate. If US investors are not invested in international stock markets in any given period, they will invest in the US risk-free rate.
The Predictive Power of OVX
The paper displays everything at monthly frequencies. The authors start by testing whether stock market returns over the previous month can returns in the following month, for all countries. Similarly, they test whether OVX changes over the past month can predict future returns.
It turns out they can. The regression outputs show that the relation between today’s stock returns and oil price uncertainty is negative. Specifically, returns are on average 1% lower per month when the one-month lagged OVX change is positive. In other words, more oil-price volatility negatively affects global stock markets.
The Trading Model
If higher lagged stock returns predict higher future stock returns, and higher oil price volatility predicts lower future stock returns, then a trading model must reflect this. The strategy suggested in the paper is as follows:
Take a long (short) position in a given month if the past monthly stock market excess return is positive (negative) and that of the OVX change is negative (positive). Otherwise, hold a risk-free asset – i.e., the one-month US interest rate.
The portfolio is then an equal-weighted average of all 55 countries, meaning excess returns over the past month are a function of equity performances in all countries.
As a benchmark, the authors use a buy-&-hold strategy which invests equally in all countries and maintains a long position for the holding period, which is one month. Another benchmark is a time-series momentum model that is only conditional on stock market returns.
Performance
The results from following these strategies from May 2007 until August 2021 show a clear winner (Chart 1).
Investing $1.00 in a standard buy-and-hold strategy would have yielded $1.62, compared with $3.61 for the cross-asset time-series model and $2.26 for the single-asset time series model. For the cross-asset time-series model, this translates into a mean excess return of 0.79% per month (9.90% per year) and a monthly SR of 0.175.
The results are for a portfolio covering all countries. At a country-level, the Sharpe Ratios for the cross-asset model are nearly all superior to the single-asset one (Chart 2).
A US Perspective
The model applied only to the US also boasts impressive numbers. Up until April 2020, the cross-asset model delivered annualised returns twice as high as a buy-and-hold strategy for the S&P500 (Chart 3). That is, from May 2007 to March 2020, conditioning a one-month lookback momentum model on oil price volatility and excess stock returns yields a 7.3% annualised return with a SR of 0.65, compared to 3.8% and 0.24 for a buy-and-hold strategy. A single-asset momentum model yielded returns of 6.6% and a SR of 0.43.
Since then, however, the model has performed notably worse than a simple buy-and-hold strategy. Even a single-asset momentum model based only on excess S&P500 returns has an annualised return of -7.0% since April 2020, compared with +13.4% for a long-only position. When conditioned on oil price volatility, the returns are even worse (-10.0%).
The poor performance of the cross-asset model, relative to the single-asset one, is due to oil price uncertainty during the recovery. Going long S&P500 requires the previous month’s stock returns to be positive and oil price volatility to decrease. In six of the 12 months in 2021, excess returns were positive, but oil price volatility increased. As a result, the model missed out on much of the recovery in equities during that period.
Over the last three months, however, the model recorded excess returns of +1%, despite the overall S&P500 being down 5%. For November, the model is giving a ‘buy’ signal.
Across all countries, the authors check what type of oil shocks the trading model may and may not capture. They find that aggregate supply and oil-specific demand shocks have a negative impact on the trading model. In other words, the trading model performs worse when there is either an unexpected increase in oil production or an unexpected increase in the precautionary demand for crude oil, i.e., an increase in oil inventories.
The ‘Smile’ in Momentum
In the seminal paper on time-series momentum models, Moskowitz and co. documented a smile-shaped pattern when plotting a momentum model’s performance against stock market returns. This is because momentum strategies usually work better under extreme market conditions.
The paper also documents such a smile-shaped relationship for their cross-asset momentum model (Chart 4). If anything, it appears to be more pronounced in both the positive and negative domains, relative to a standard single-asset momentum model.
From a portfolio diversification perspective, the results mean that the cross-asset model is more valuable because it offers both higher returns when market returns are either positive or negative.
A Predictor of Economic Growth?
As a final step, the authors look at whether the strategy’s performance can be linked to economic activity.
It can. Across all countries, positive stock returns and negative OVX changes are associated with better future economic prospects. Indeed, in the US model, the performance of the cross-asset time-series momentum model is a very good 10-month leading indicator of the S&P500 (Chart 5). The correlation is 0.88 from 2007 to 2022 and implies that US equities may have room to lower over the next year.
Bottom Line
Momentum models are popular among practitioners. Here, we see one way such models can be improved. The reason is that oil prices and uncertainty can have real impacts on businesses, leading to changes in the economy and stock prices. Thus, simply conditioning on oil price volatility can potentially deliver higher excess returns in the long run. Crucially, the strategy also offers higher returns in more negative states of the economy and gives leading medium-term (not just short-term) insights into the future direction of equities.
Sam van de Schootbrugge is a Macro Research Analyst at Macro Hive, currently completing his PhD in international finance. He has a master’s degree in economic research from the University of Cambridge and has worked in research roles for over 3 years in both the public and private sector.