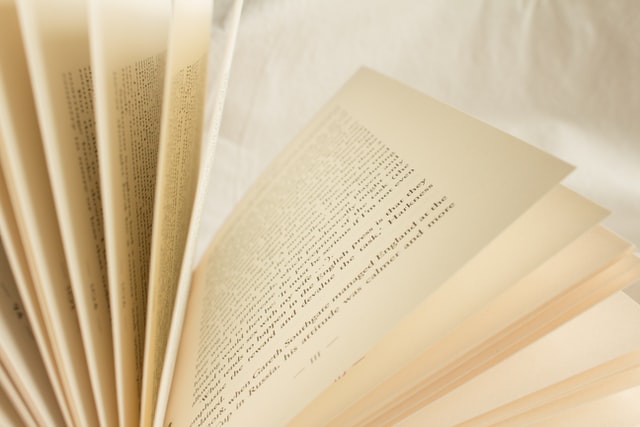
Monetary Policy & Inflation | US
Monetary Policy & Inflation | US
This article is only available to Macro Hive subscribers. Sign-up to receive world-class macro analysis with a daily curated newsletter, podcast, original content from award-winning researchers, cross market strategy, equity insights, trade ideas, crypto flow frameworks, academic paper summaries, explanation and analysis of market-moving events, community investor chat room, and more.
The Beige Book is a Fed publication released eight times a year before FOMC meetings. Federal Reserve Banks across 12 districts of the US gather anecdotal information on current economic conditions through interviews with ‘key business contacts, economists, market experts, and other sources. The Beige Book distils the economic views by district and provides a national summary.
Since the information contained in the Beige Book influences Fed decisions, it makes sense for investors to read reach report diligently. However, a typical Beige Book report contains over 10,000 words, which many find daunting. We therefore use machine learning to turn the report into a sentiment score.
In machine learning, one way to navigate a sea of text and audio-based information is with natural language processing (NLP) techniques. The goal of NLP is to understand textual data to contextualise and extract useful information within it. One application of NLP is sentiment analysis. Sentiment analysis aims to classify whether the opinion expressed in a text is positive or negative (or neutral).
We focus on the Beige Book and derive a sentiment score by looking at the proportion of positive and negative words in each report. We calculate a raw sentiment score at a district level. Then we aggregate (equally weighted district level average followed by smoothing and detrending) these into an overall sentiment index. We can do this in real-time as soon as the report is released. Charts 4 and 5 show a comparison of our sentiment score to the US ISM PMI and the University of Michigan Consumer Sentiment Index for the US.
Spring sale - Prime Membership only £3 for 3 months! Get trade ideas and macro insights now
Your subscription has been successfully canceled.
Discount Applied - Your subscription has now updated with Coupon and from next payment Discount will be applied.