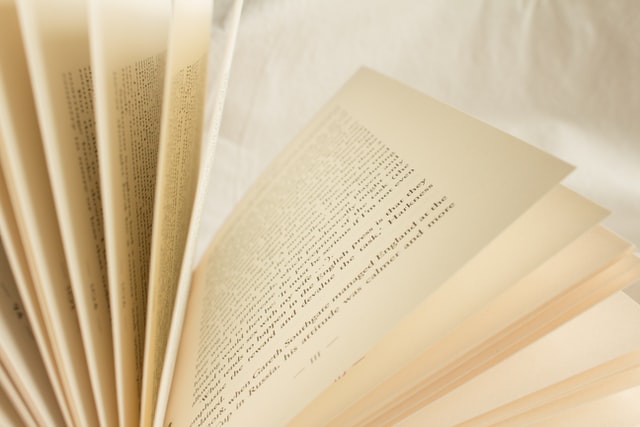
Monetary Policy & Inflation | US
Monetary Policy & Inflation | US
This article is only available to Macro Hive subscribers. Sign-up to receive world-class macro analysis with a daily curated newsletter, podcast, original content from award-winning researchers, cross market strategy, equity insights, trade ideas, crypto flow frameworks, academic paper summaries, explanation and analysis of market-moving events, community investor chat room, and more.
We use machine learning to convert the Fed’s text-heavy Beige Book into a sentiment score. The latest Beige Book report released on 13 July shows a decrease in sentiment from the last report on 1 June (Chart 1). The sentiment remains near the lows of the 2 March release. This marks the first decrease in sentiment since the 2 March release, which was followed by two increases in sentiment (20 April and 1 June reports).
Here is a summary of the latest developments:
This small change in sentiment could reflect that the economy is currently going through an air pocket caused by fiscal consolidation, a strong dollar and a small inventory cycle that are likely to get resolved by end-year (see US Growth: Air Pocket Not Crash).
In machine learning, one way to navigate a sea of text and audio-based information is with natural language processing (NLP) techniques. The goal of NLP is to understand textual data to contextualise and extract useful information within it. One application of NLP is sentiment analysis. Sentiment analysis aims to classify whether the opinion expressed in a text is positive or negative (or neutral).
We focus on the Beige Bookand derive a sentiment score by looking at the proportion of positive and negative words in each report. We calculate a raw sentiment score at a district level. Then we aggregate (equally weighted district level average followed by smoothing and detrending) these into an overall sentiment index. We can do this in real-time as soon as the report is released. Charts 4 and 5 show a comparison of our sentiment score to the US ISM PMI and the University of Michigan Consumer Sentiment Index for the US.
Spring sale - Prime Membership only £3 for 3 months! Get trade ideas and macro insights now
Your subscription has been successfully canceled.
Discount Applied - Your subscription has now updated with Coupon and from next payment Discount will be applied.