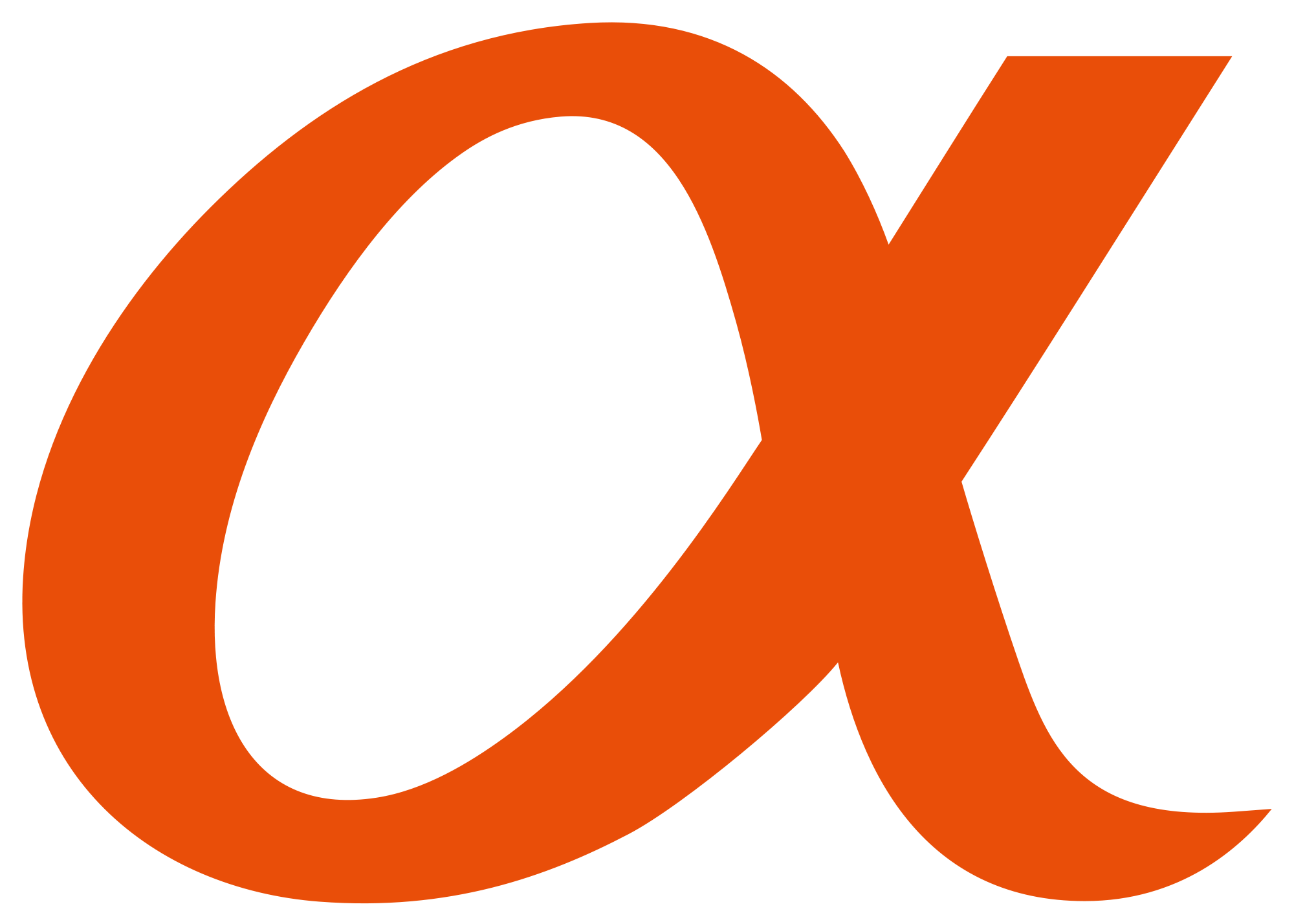
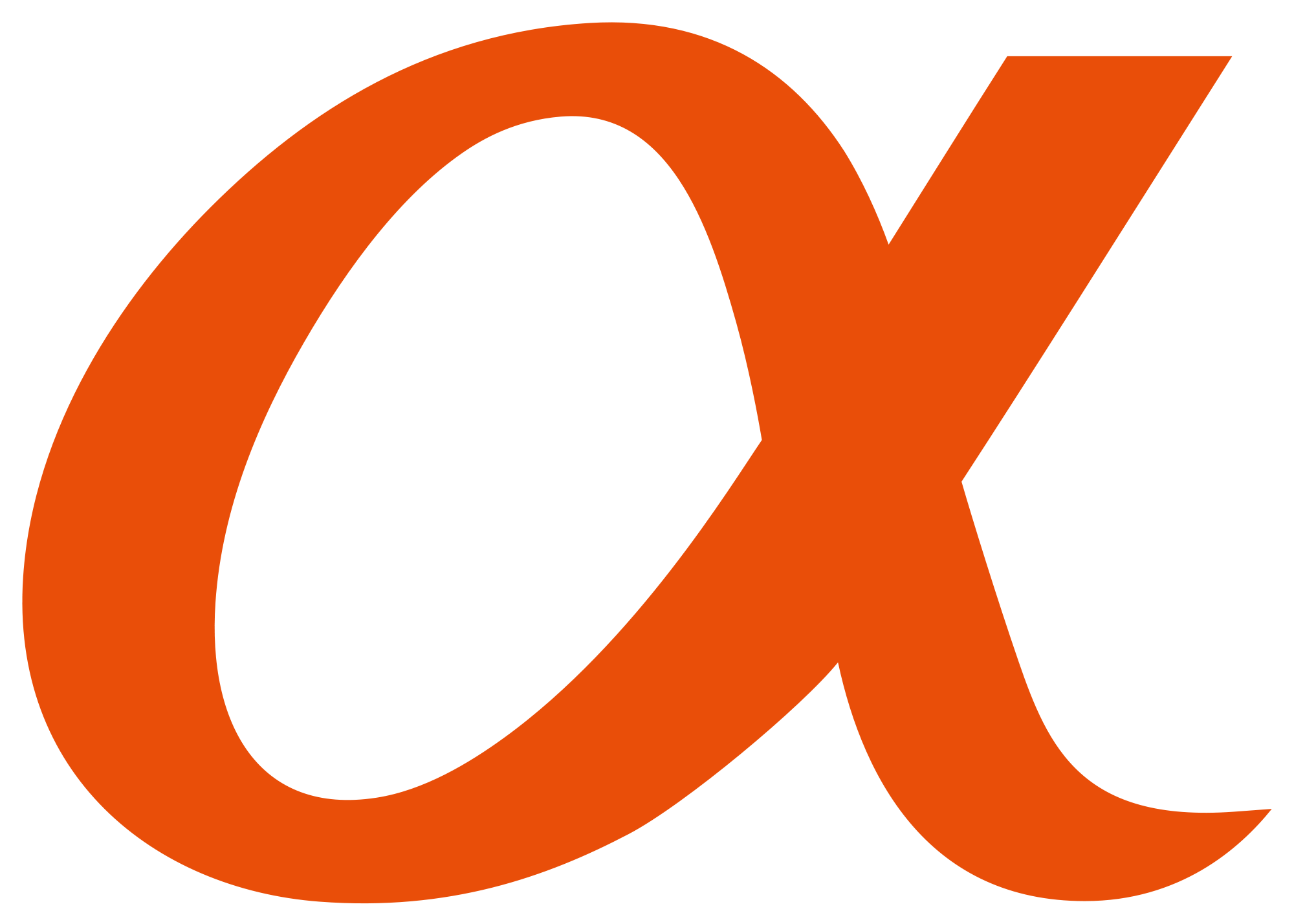
In recent years the availability of new alternative datasets for financial markets has expanded enormously, potentially giving investors an informational edge. These can range from consumer transaction data to satellite data, and even on occasion news data. Unlike traditional market data, these new sets of information can come in formats more challenging to analyze – such as text or images. Making sense of it all often requires a large investment of time to structure such datasets into more readily accessible formats, such as numerical time series…
This article is only available to Macro Hive subscribers. Sign-up to receive world-class macro analysis with a daily curated newsletter, podcast, original content from award-winning researchers, cross market strategy, equity insights, trade ideas, crypto flow frameworks, academic paper summaries, explanation and analysis of market-moving events, community investor chat room, and more.
In recent years the availability of new alternative datasets for financial markets has expanded enormously, potentially giving investors an informational edge. These can range from consumer transaction data to satellite data, and even on occasion news data. Unlike traditional market data, these new sets of information can come in formats more challenging to analyze – such as text or images. Making sense of it all often requires a large investment of time to structure such datasets into more readily accessible formats, such as numerical time series.
Moreover, to extract the maximum values from them, it is insufficient simply to collect them together into a machine learning model so as to spit out buy or sell signals. Instead, research into such datasets needs to be guided by the right questions. It also needs to be able to combine the various datasets successfully to produce meaningful answers. This can be a tricky task.
The Micro Case
For example, say we are trying to forecast earnings per share of a specific retailer ahead of its official announcement. We can use alternative data to tackle this from several angles. First, we might obtain car count data gathered from satellite imagery. This would allow tracking the occupancy of car parks attached to a retailer to gain an estimate of the number of customers visiting it. Whilst unreliable when used alone, we can augment this information with mobile phone location data to estimate actual foot traffic within those stores. We can even dig down further and use consumer transaction data to estimate how much each customer is spending at the store. By carefully combining these datasets, all of which are available on a high-frequency basis, we can fairly securely incorporate such observations into our forecast for earnings per share.
The Macro Case
Alternative datasets can also be used on a macro level. We can, for instance, construct indices to measure sentiment for Federal Reserve communications (see Figure 1). Such an index would ingest a large number of communications from the Fed, sourced from statements, minutes, and even transcribed speeches. Natural language processing could then be applied to the text to gauge Fed sentiment. Such indices are relatively correlated to changes in US Treasury yields (see chart). This becomes particularly interesting when the market (as reflected by yields) is saying one thing, while simultaneously the Fed (as reflected in the sentiment index) is saying another. Currently, yields appear too low compared to Fed sentiment.
New Avenues
Other alternative datasets for the macro world would include an examination of the volume of Bloomberg News articles related to upcoming FOMC meetings so as to understand better any potential volatility surrounding them. We can also use flow data from CLS (a settlement service for foreign exchange markets) to understand price action on an intraday basis, or even use consumer transaction data to construct estimates of national retail sales statistics.
Ultimately, for those of us involved in financial markets, restricting ourselves to traditional datasets means missing out on the bigger picture. More data can help to give us more insights, but we first have to know how to utilize it.
Chart 1: Fed Communication Score and US Yields
Source: Cuemarco, Fed
Saeed Amen is the founder of Cuemacro, which provides consulting services for investors involved in systematic trading. He is the author of Trading Thalesians: What the ancient world can teach us about trading today (Palgrave Macmillan) and the co-author of The Book of Alternative Data (Wiley), forthcoming in 2020.
(The commentary contained in the above article does not constitute an offer or a solicitation, or a recommendation to implement or liquidate an investment or to carry out any other transaction. It should not be used as a basis for any investment decision or other decision. Any investment decision should be based on appropriate professional advice specific to your needs.)